It’s fair to say that 2024 has seen something of a revolution in localization, with the entire function taking a big step up in the minds of enterprise leadership. Rather than a backstage player, localization is receiving much greater recognition as a strategic powerhouse driving market expansion, cultural connection, and brand identity on an unprecedented scale.
Change, of course, brings opportunity, but also a level of uncertainty. As we all sit down to reflect on the past year, and plan for the year ahead, this has lent something of a philosophical edge to discussions, and this was certainly true in Lingoport’s recent webinar on trends for 2025.
Lingoport CEO Adam Asnes welcomed a panel of seasoned experts to explore practical, technical, and the more esoteric impacts on the language industry, Renato Beninatto, Chairman and Co-Founder of Nimdzi, joined Marco Trombetti, Co-Founder and CEO of Translated, Motorola’s Senior Technical Project Manager Luz Pineda, and Phrase CEO Georg Ell.
Here, we’ve gathered some of the most interesting points and comments from a wide ranging discussion that covered the entire localization ecosystem.
Moving beyond traditional constraints
From the outset, there was an acknowledgment that localization is more than a mechanical process of cost and turnaround times. “We’ve traditionally looked at localization through the triangle of quality, time, and cost,” Renato noted. “But now we’re moving beyond these constraints into unchartered territories.”
The panelists urged participants to view localization not merely as a “final polishing” step, but as a strategic function that can accelerate global reach, unlock new markets, and inform a brand’s global voice.
While cutting costs and speeding up delivery will always matter, the real opportunity lies in using AI to translate and transform content at a scale and depth previously unimaginable.
Automating quality evaluation and moving QA upstream
A key theme was how AI, particularly large language models (LLMs), can help push quality assurance further “upstream.”
Luz Pineda described Motorola’s current push toward leveraging AI-driven linguistic checks at the very start of the localization lifecycle. Traditionally, organizations waited until late in the process—after multiple handoffs—before running linguistic quality assurance.
Now, AI can instantly assess translations, flag potential errors, and even ensure they fit tight UI space constraints before a human reviewer lifts a finger.
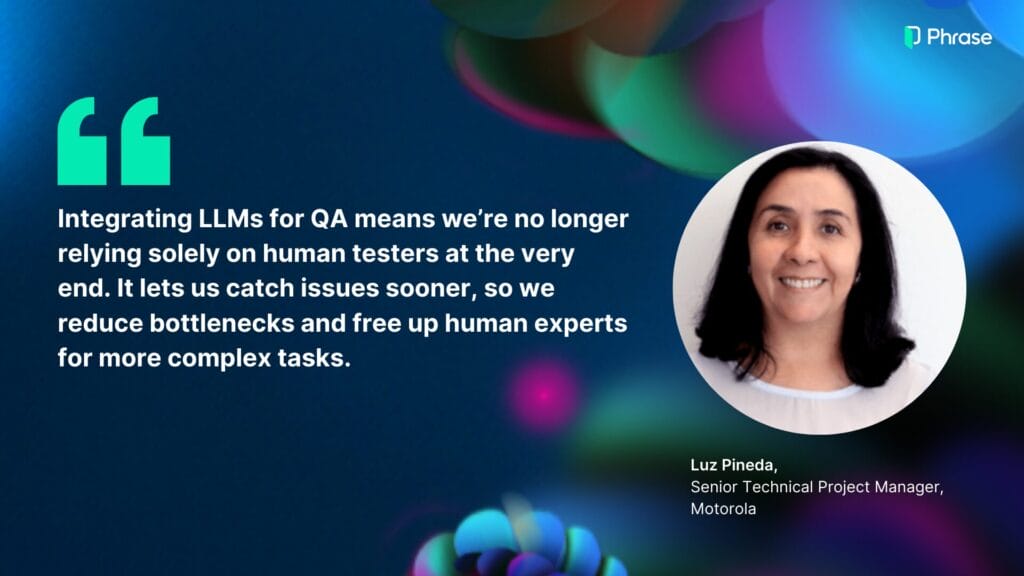
This isn’t a trivial point. Luz credited insights from experts like Marina Panchava on prompt engineering, learning that controlling and shaping LLM behavior requires a careful combination of metadata, instructions, and linguistic assets.
You cannot do this in a month. It’s an iterative process that requires careful collaboration, the right tools, and a willingness to learn. From metadata handling to workflow adjustments, every step builds toward a more seamless integration of AI-driven quality assurance.” – Luz Pineda, Motorol
The next generation of translation models
Among the most intriguing innovations discussed was LARA, the new architecture introduced by Marco Trombetti and his team at Translated.
LARA represents a fusion of powerful neural machine translation (NMT) systems and large language model capabilities, moving beyond sentence-by-sentence translation to document-level, context-rich processing.
Traditionally, MT systems worked in isolation, churning out segment translations without a sense of broader narrative or brand voice. LARA flips that script.
“We’ve combined the fluency and flexibility of language models with the accuracy of specialized translation models,” Marco explained. “Now the model isn’t just translating; it’s taking in full documents, understanding context, and even asking for clarification if needed.”
The ultimate goal for these newer models is to reduce errors down to negligible levels and achieve a form of linguistic “singularity”—a point at which machine translations are reliably as good as, if not better than, the average professional translator for certain content types.
In one example, Marco noted how LARA has pushed error rates from around 12 errors per 1,000 words in typical MT models to just 2.5, approaching the best human professionals.
It’s important to note this is a stepping stone however, not an endpoint, as Marco explained.
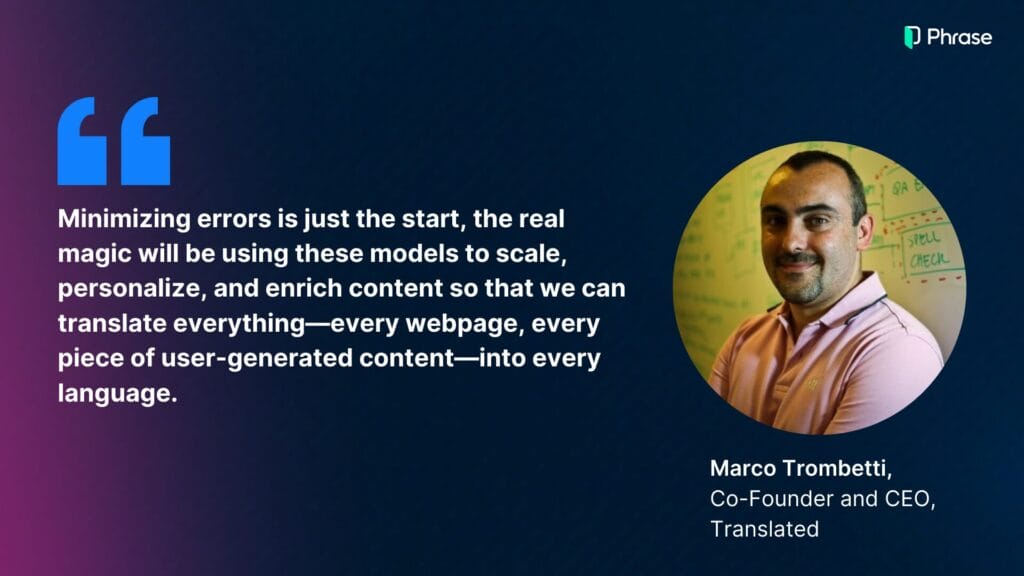
Beyond cost: What is AI in localization really for?
Early AI discussions in localization often centered on cost savings. But the panel was unanimous in seeing beyond mere efficiency.
“It’s not just about saving money; it’s about doing more with the resources we have,” said Marco. AI can help teams handle larger volumes, shorten turnaround times, and localize content that previously would have been out of scope or budget.
Luz added that Motorola uses AI to scale its localization efforts, allowing the same team to handle more repositories and projects while maintaining—if not improving—quality standards.
“By scaling, we create more opportunities, we go from translating a fraction of the world’s content to almost all of it, democratizing access to information across linguistic and cultural borders.”
AI as Co-Pilot: The human factor
If machines are getting smarter, faster, and more context-aware, where does that leave human translators, project managers, and linguists?
Georg Ell offered a reassuring vision: AI doesn’t eliminate human roles—it reshapes them. The future he imagines is one where AI and humans work in tandem, each complementing the other’s strengths.
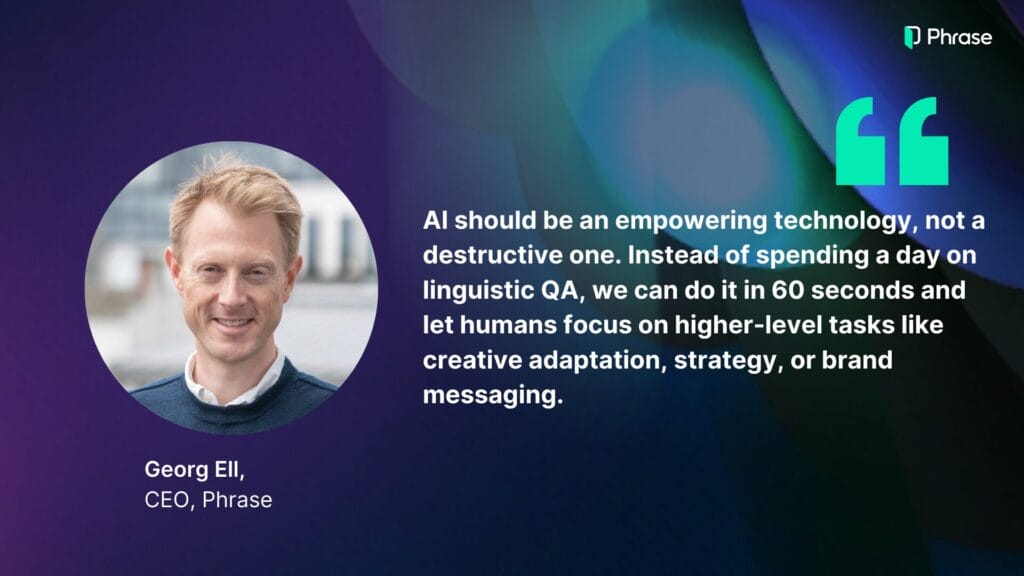
This co-pilot model aligns with the philosophical shift happening in localization: move humans away from monotonous error-spotting and toward tasks that require empathy, cultural understanding, and editorial judgment—areas where technology, no matter how advanced, struggles to emulate the human touch.
Leveraging context: UI constraints and cultural nuances
Taking a deeper look at the granular applications of new technology, Luz described how a single translated string might need to fit into a button on a mobile UI.
The challenge is not only to ensure correctness, but also to adapt that translation so it doesn’t overflow or get truncated. Integrating metadata—like character limits or style guides—into the prompt can guide the LLM to produce translations that respect these constraints right from the start, solving a host of traditional problems by referencing additional data options.
Similarly, Marco spoke about document-level translation and how LLMs can consider brand terminology, tone of voice, and even demographic data to produce content that resonates.
Georg offered the idea of hyper-personalization: dynamically adjusting a website’s tone and message based on current events, cultural sensitivities, or individual user preferences:
We’re talking about changing the content on-the-fly. If a significant event happens in a particular country, AI could instantly shift the tone of the localized content to be more empathetic, respectful, or informative.
– Georg Ell, CEO, Phrase
Language, intelligence, and trust
The discussion wasn’t just about technology and process. As Marco mentioned, we often assume human intelligence and linguistic ability are fixed benchmarks. But what if they aren’t?
“Language is the most human thing we have, but there’s no law in physics that says our brains are the pinnacle of intelligence. If we can build machines smarter than us in certain areas, how does that change our understanding of language and communication?”
This philosophical thread touches on trust and authenticity: how do we trust AI-driven translations if they become indistinguishable—or even superior—to human work?
The implication is that just as we continue to evolve the translations themselves, we also need to make sure measures of quality and impact keep up. Instead of fixating on small errors, we should look at engagement, understanding, and the seamlessness of experience.
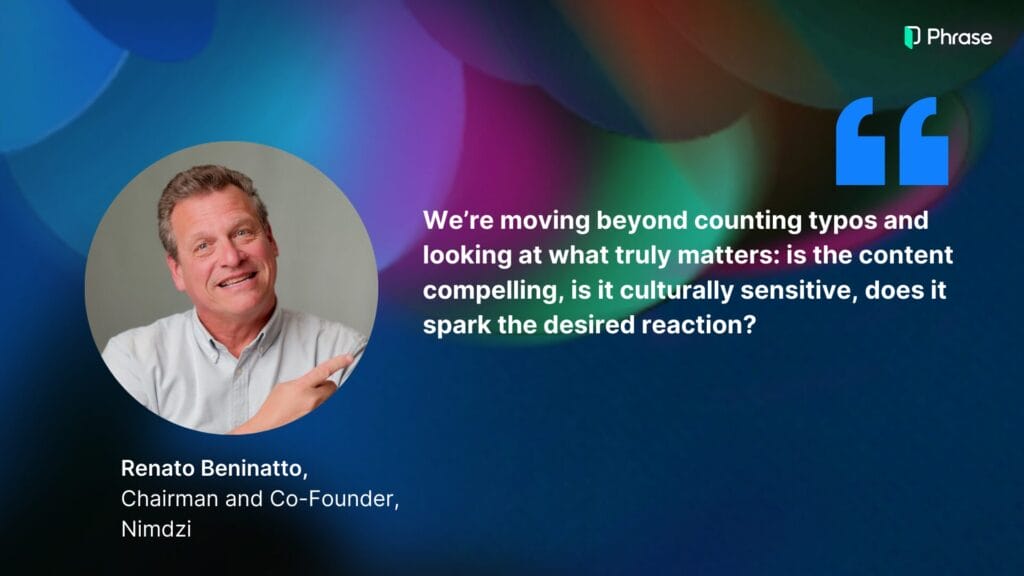
The roots of modern AI translation
The panel also took time to discuss ‘how we got here’, looking at some of the historical and technical underpinnings of modern AI systems.
The transformer architecture—pioneered through research in the language field—has been the backbone of many breakthroughs in machine translation and LLMs.
The discussion touched on the irony of localization professionals viewing AI advances as external forces.
In reality, research in machine translation and language modeling helped inspire and shape these very transformer-based architectures.
We invented the transformer in this industry, this technology didn’t drop from the sky. It grew out of attempts to solve our core problems. We should embrace it as an integral part of our toolkit.
– Marco Trombetti, Co-Founder and CEO, Translated
We invented the transformer in this industry, this technology didn’t drop from the sky. It grew out of attempts to solve our core problems. We should embrace it as an integral part of our toolkit.”—Marco
Embracing the future
There are still hurdles to overcome. Talent shortages in AI-savvy localization roles, infrastructure costs for training and fine-tuning models, and the need for careful prompt engineering are all barriers.
Despite this, the panel are optimistic: These are challenges to be managed, not showstoppers.
The future of localization lies in symbiosis, humans and AI collaborating to drive unprecedented growth and innovation, delivering experiences that resonate across languages and cultures.
– Georg Ell, CEO, Phrase
2025 and beyond: A time of boundless potential
The webinar closed on a note of possibility.
The industry is not facing an existential threat from AI; it’s seizing a new horizon. LLMs, new models, and evolving language industry ecosystems are giving localization professionals unprecedented control, scale, and nuance.
We can elevate localization from a back-office function to a front-line strategic driver. The tools are here. The world is waiting.
– Renato Beninatto, Chairman and Co-Founder, Nimdzi