Phrase and beyond Translation management
Understanding QPS and Its Expected Savings and ROI
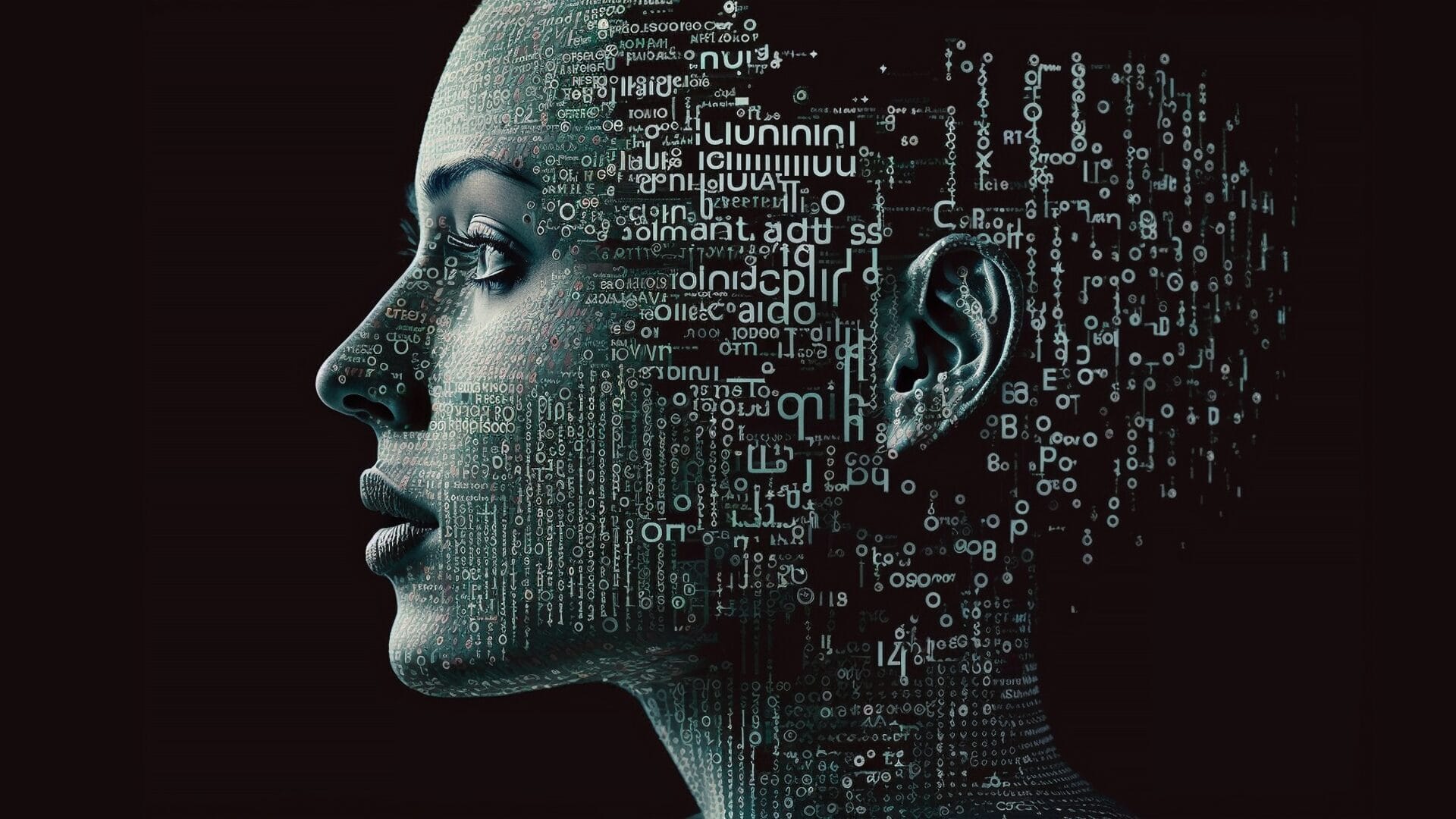
In my previous article in this blog series, I described Phrase Quality Performance Score (QPS), and how it is designed to provide translation quality visibility across the various different workflow steps of the Phrase Localization Platform.
A key rationale behind the need for such translation quality visibility is that it unlocks and enables hyperautomation.
This allows our customers to maximize the value of Machine Translation (MT) while strictly optimizing for the right tradeoff balance between automation and level of quality risk.
The goal is to enable organizations to automatically detect and address low-quality translations efficiently within the Phrase translation workflow process, minimizing the need for extensive human intervention.
Phrase QPS assigns quality scores at the segment level, which are then aggregated to the document and job level. Routing and decision-points can then be implemented within the workflow to support two major complementary decisions:
- At the job-level: is a translated job of sufficient quality to be completed without further human editing or review?
- At the segment-level, for jobs that are sent to human editing: which segments are of sufficiently high quality and can be “blocked” from human editing and correction?
Today, we’d like to focus on the second use case – segment-level QPS-based editing.
Most concretely, we were curious to investigate and answer the question of how much editing time (and thus cost) could be saved by restricting editors to focus exclusively on editing lower-quality segments, as identified and determined using QPS.
For any given job, the answer to this question is a function of the QPS quality profile (the distribution of QPS scores) of the various segments in the job.
Of course, the exact quality profile of any job that is pre-translated by MT and then evaluated using QPS depends on many factors.
First and foremost it depends on whether the MT system used is generally accurate and well-suited for translating the specific job in question to begin with.
But to answer the potential savings question, we’ve conducted a series of simulation studies in partnership with some of our major customers.
The setup was the following:
- We first identified a sizable collection of jobs from a domain and use-case typical for the customer, which had already been fully pre-translated by MT and then post-edited on the Phrase Platform.
- We next scored the MT-generated translations for all segments within this collection using QPS.
- We then ranked the entire collection of segments based on their QPS score.
- Finally, we analyzed the profile of this collection, by looking at the fraction of segments that would be excluded from human editing based on various QPS quality thresholds.
Below we highlight the results obtained from one representative instance of such an analysis, from a customer in the technology domain.
The project consisted of around 3,500 jobs, totaling about 35,000 segments which amounted to approximately 457,000 words. The language-pair was English-to-German.
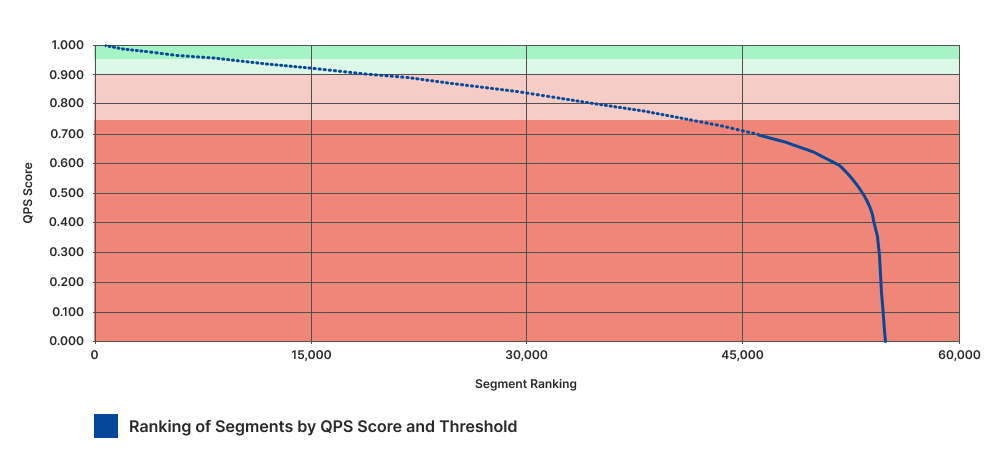
As can be seen in Fig. 1, MT quality, as determined by QPS, was generally very high.
Ranking the segments by their QPS score indicates that QPS scores drop very slowly for the vast majority of the content
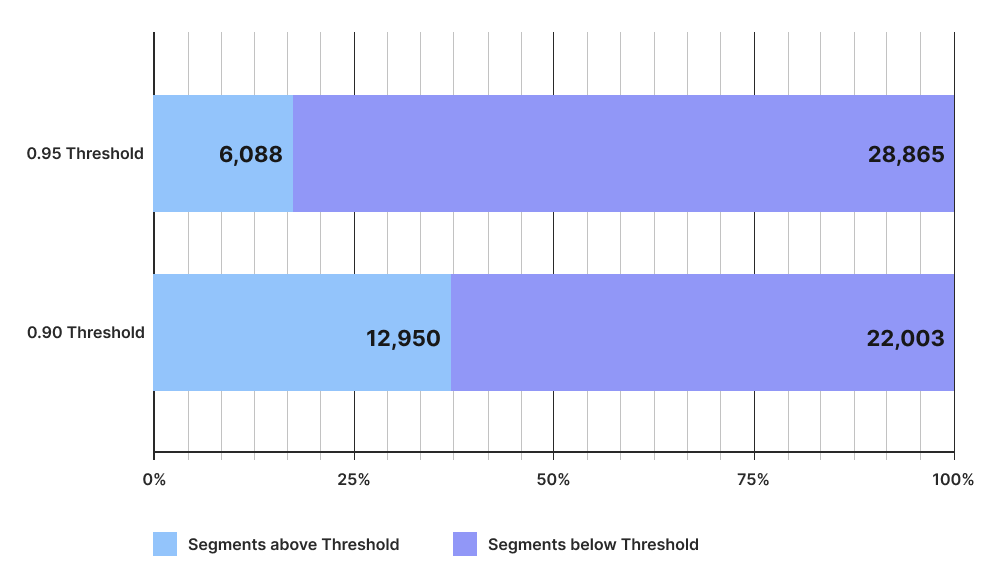
As can be seen in Fig.2, if we split the content based on a QPS score threshold, 17% of segments have a QPS score of 95 or higher, while 37% of segments have a QPS score of 90 or higher. Or observed from the other end of the spectrum, only 20% of segments have a QPS score of 75 or lower.
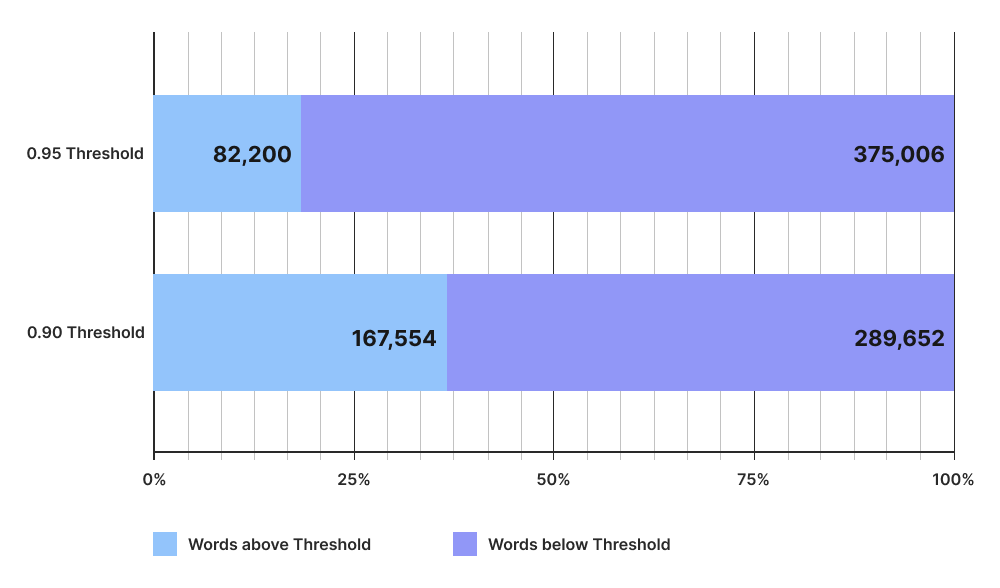
Fig. 3, shows that the word distribution, based on the same QPS thresholds, is very similar.
Segments (and the words within them) that score above threshold then become candidates for blocking from human editing.
This allows us to then calculate the expected savings from foregoing human editing on high-quality MT segments, based on any selected QPS score threshold.
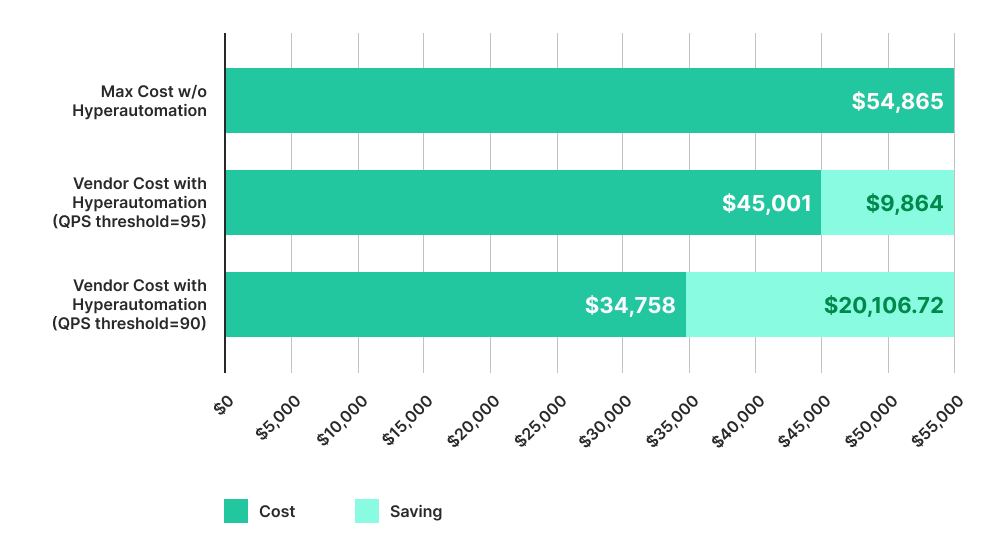
Fig. 4 highlights the expected savings from setting the editing threshold at a score of either 95 or 90, assuming an average MT post-editing cost of $0.12 per word.
While the distribution profile and potential savings analysis, as presented above, will of course vary across projects, domains, content-types and language-pairs, the results above are representative of the significant savings that can be expected in many enterprise scenarios with today’s state-of-the-art MT.
QPS provides the information necessary in order to allow customers to dynamically configure the tradeoff they desire and need between quality and cost across their translation projects.
Decisions can now be made by either setting quality thresholds for human editing, or alternatively, by setting a target cost for a project, and then directing only the fraction of lowest-quality segments that should be edited in order to meet that target cost.
Phrase QPS: providing visibility into translation quality
In conclusion, the Phrase Quality Performance Score (Phrase QPS) enhances translation workflows by providing visibility into translation quality, enabling a balance between MT efficiency and quality control.
By focusing human editing on lower-quality segments identified by Phrase QPS, organizations can achieve significant cost savings and greater efficiency. This allows precise targeting of human editing efforts, reducing time and expense.
Ultimately, Phrase QPS helps streamline translation processes, maximize ROI, and ensure optimal use of resources in translation and localization efforts.
Speak with an expert
Want to learn how our solutions can help you unlock global opportunity? We’d be happy to show you around the Phrase Localization Platform and answer any questions you may have.