While Machine Translation has been with us in one form or another for quite some time now, it’s easy to see why some organizations may be uncertain about embracing it.
You may already have your own MT solutions that aren’t quite performing as you need them to, but worry that adopting a multi-provider platform will be too expensive. There’s an ongoing concern over quality, and a skepticism that MT will ever be able to match the precision of human-curated Translation Memories (TMs).
Concerns about privacy and security also loom large for industries handling sensitive content, and there’s still the impression in many areas that “MT just isn’t good enough.”
But as global content needs continue to surge, modern AI-driven MT technologies offer some compelling advantages in speed, scalability, and cost efficiency. In this post, I wanted to look at some of the advantages and key use cases for MT, and address some of the areas where it can compliment—or even outperform—more traditional Translation Memory-based processes.
1. Coverage and adaptability for new content
Traditional Translation Memories will only contain entries for text segments that have been translated and approved in the past. While this is a great way to maintain consistency, a TM will struggle to find a meaningful match when it encounters a new segment, or a text with low similarity to existing text. This can lead to process delays, as linguists are forced to step in and translate content from scratch.
Modern Machine Translation systems, especially Neural Machine Translation (NMT) and Large Language Model (LLM)-Based MT, can generate translations for entirely new or drastically different content. The MT model’s internal parameters capture broad linguistic patterns, so it can produce a high quality first-draft translation even if that segment has never been encountered before. Having a basic version to work from can massively speed up linguists’ workflows, even for complex documents.
Instead of encountering a “0% match” or a low fuzzy match in the Translation Memory, translators can begin with a machine-generated draft, providing a helpful starting point. This significantly reduces the time and effort required, particularly for high-volume or time-sensitive projects, enabling linguists to focus on refining the translation rather than starting from scratch.
In addition to this, advancements in intelligent language technology mean tools (Including our own Phrase Language AI) can now dynamically select the best MT engine for a given task. This gives more flexibility and a greater range of contextual translation options than a TM alone.
By evaluating factors like content type, domain, and language pair, and drawing on historical performance data (e.g., post-editing effort), you can ensure you’re using the most effective engine for the task at hand.
By tailoring the MT engine to the specific needs of the content, this approach minimizes the time spent on manual translation adjustments and maximizes the benefits of both TMs and MT systems, creating a more efficient and reliable process.
2. Speed and scalability
Translation Memories provide immediate time and cost-savings for translation jobs which require similar segments to be repeated often. This is great for content like user manuals or technical documentation, which often involve repeated segments, step-by-step instructions, or product descriptions, across multiple documents or product versions.
However, if there is a large volume of new or particularly diverse content, TMs alone cannot handle the sheer velocity of updates—every non-matching segment still relies on manual human translation.
Machine Translation engines can process millions of words in a very short time, providing a draft translation for each segment instantaneously. This is particularly useful if you are dealing with:
- User-generated content (e.g., support tickets, user reviews)
- Dynamic content (e.g., ecommerce product descriptions that constantly change)
- Bulk updates (large-scale websites or knowledge-base updates)
Again, this enhances your operational efficiency, providing faster turnaround times, so that the humans in the loop can focus more of their time on post-editing, and less on re-translating text.
There’s also the obvious advantage of real-time, or near-real-time translation, making use cases like cross-platform updates, or live chat more manageable, and more effective.
Handling translation at scale requires more than just speed—it demands reliability and adaptability. As I mentioned earlier, modern solutions now combine multiple Machine Translation engines with intelligent selection mechanisms.
This approach means a consistent stream of high-quality translations, even when managing much higher volumes of content. Being able to aggregate engines this way means that the entire process becomes vastly more scalable, without affecting security, or accuracy.
3. Continuous improvement and adaptation
A Translation Memory is essentially a repository. It is a secure, robust way to make sure everything being translated adheres to certain pre-defined conditions. While it can be updated with new segments, it doesn’t “learn” from corrections in the way an LLM or NMT might. This means that if a term has been translated incorrectly or out-of-context in the TM, a human will need to review, spot, and fix the translation every time it happens.
In contrast, Machine Translation solutions can be fine-tuned using domain or brand-specific data. This means that adaptations can be continuously improved, allowing for ongoing updates to your terminology. Many modern MT solutions, especially neural and large language models, can be fine-tuned on domain-specific or brand-specific data. This means they can adapt over time, incorporating corrections and updated terminology to reduce errors in subsequent translations.
As updates and corrections are applied, the MT engine becomes increasingly aligned with specialized vocabulary, industry jargon, and consistent brand language.
This ongoing refinement can be especially useful in areas like medical, legal, and technical content, where accuracy is essential and new terminology emerges frequently. Because the engine applies lessons learned from post-editing efforts, each completed translation project contributes to a more robust and reliable system. Over time, the result is a steady improvement in translation quality, and reduced post-editing workloads.
4. Integration with existing workflows and technology
Despite the advantages of MT, TMs remain an essential tool—particularly for legal disclaimers, stable UI strings, or brand slogans that must remain consistent. A common approach is to rely on the TM first, especially for any segments that are repeated or have high-fuzzy matches.
This is useful for material like user guides, or well-established UI strings that must stay consistent across every translation. If the TM doesn’t provide a good match, an MT engine can then be used to fill in the gaps, ensuring that both previously approved translations and newly generated ones work together.
Modern MT platforms are also designed to integrate seamlessly with Translation Management Systems (TMS) and Computer-Assisted Translation (CAT) tools.
Because these platforms are modular, they can be set up to run only after the TM has produced its best match, ensuring that the final translation cycle remains smooth. This hybrid approach means you can preserve the value of the investments you’ve already made in building and maintaining a TM, while still benefiting from the flexibility of automated translation.
As a result, you can avoid disruptions when incorporating MT into your existing setup, and maintain the option to use TMs for pieces of content that absolutely must remain unaltered.
In many cases, glossaries and terminology databases can be used alongside the MT engine to maintain consistent brand voice and specialized vocabulary.
By enforcing the use of certain terms during the machine translation process, companies can ensure that crucial brand or industry-specific language remains accurate, even when the system is generating translations in real time.
When TMs, MT, and glossaries work together, it creates a more fluid and efficient environment, allowing linguists and project managers to focus on fine-tuning translations rather than performing repetitive manual tasks.
5. Quality control and terminology consistency
Quality control is a cornerstone of any successful translation strategy, and both Translation Memories and modern Machine Translation engines address this need in different ways.
When a TM is properly curated, each stored segment has already been vetted and approved by a human translator, making it a reliable source of consistent reuse. However, TMs can become bloated or contain duplicate or conflicting entries if they aren’t regularly maintained, which can undermine the consistency and accuracy they’re meant to provide.
On the other hand, current MT engines are capable of interpreting context beyond single sentences, and this broader understanding can significantly enhance overall coherence and fluency.
By ensuring that key terms are correctly translated from the beginning, MT can decrease repetitive corrections and streamline post-editing.
These improvements matter most when you’re dealing with repeated phrases, or highly technical information. Mistakes here disrupt the reader’s experience, and increase workload for editors, who need to correct the same errors repeatedly.
To address these challenges at scale, many modern platforms now include automated quality assurance (QA) mechanisms. These tools can automatically scan translations for inconsistencies, errors, and deviations from approved terminology databases or style guides.
Rather than relying entirely on human reviewers, automated QA provides real-time alerts and scoring systems that help identify problem areas before translations reach the final stage.
Additionally, AI-powered quality metrics—such as hLepor, BLEU, or COMET—can evaluate the fluency and accuracy of MT outputs based on previous human-reviewed content. When combined with centralized glossaries and linguistic rule enforcement, these metrics make it easier to catch discrepancies early, reducing the burden on post-editors and improving consistency across projects.
All of these measures—combining TMs for proven translations, leveraging MT for advanced linguistic capabilities, and integrating automated QA checks and real-time terminology enforcement—work together to raise the overall quality and reliability of your translation projects.
By reducing manual effort, surfacing issues earlier in the workflow, and ensuring uniform terminology usage, organizations can achieve more polished results in less time.
6. Cost efficiency
When Translation Memories yield a high-fuzzy or 100% match, translation cost is much lower than doing a full manual translation. However, TMs do not offer significant savings for content that is either completely new or only partially similar to existing segments.
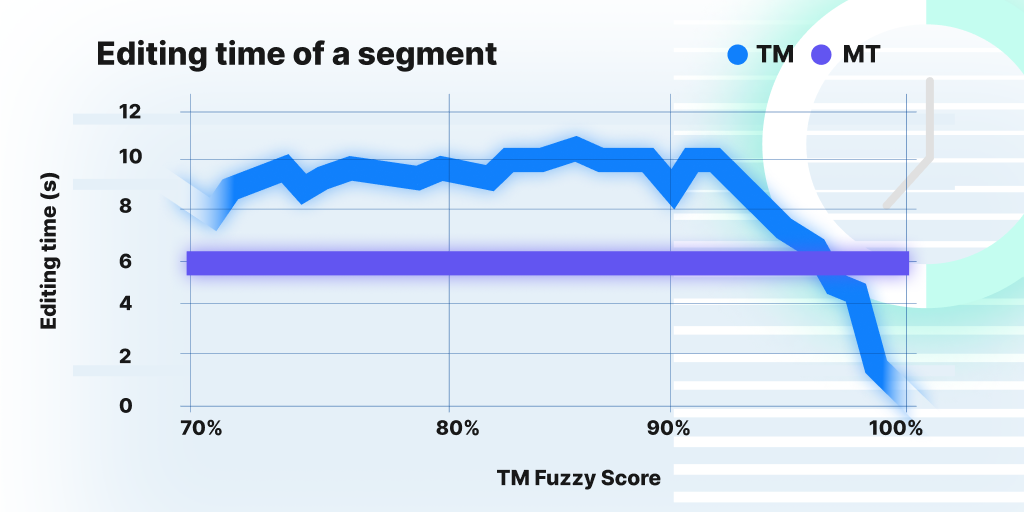
This is where Machine Translation (MT) comes in, providing an instant draft at a cost per word that is typically far lower than starting from scratch. By relying on a post-editing process to refine MT outputs, organizations can see these cost benefits add up quickly—especially when dealing with high volumes of text.
In addition to direct cost savings, this approach frees up valuable resources that can be funneled into more specialized or creative tasks.
Because MT handles the repetitive, high-volume work, organizations do not need to maintain a large pool of translators year-round, and can adapt more easily to seasonal spikes or large user-generated content streams.
Again, by training MT engines on domain-specific language assets, you improve the quality of the initial drafts over time. From a business perspective, the end result is a more efficient workflow, a streamlined budget, and a reallocation of resources toward higher-value projects that benefit most from human expertise.
7. Security and compliance
Data security is one of the more common concerns for organizations looking at Machine Translation, and rightly so—particularly if you are operating in regulated fields like finance, healthcare, or government.
Typically, Translation Memories reside in a controlled environment, making it easier to adhere to strict privacy and data protection requirements. However, some generic Machine Translation engines require content to be sent to external providers, which can raise confidentiality risks if the materials are sensitive or contain personally identifiable information.
To address these issues, modern solutions offer secure deployment options. MT services can be hosted on-premise or in cloud environments that meet rigorous standards for privacy and data handling.
In some cases, multiple MT engines can be managed under a single infrastructure, where legal and security evaluations are performed collectively, removing the need for separate compliance checks for each provider. This approach allows organizations to maintain control over how data is processed and ensures that it does not end up in publicly accessible or unprotected storage.
These measures are particularly critical when dealing with regulations like HIPAA, GDPR, or specific financial and legal statutes. Proper handling and protection of data not only mitigate the risks of breaches or leaks but also help preserve trust with clients, partners, and regulatory bodies. Ensuring that translation processes comply with these requirements is therefore an essential step toward upholding a secure and reputable operation.
8. MT advantages in the real world
The need for fast, efficient, and scalable translation solutions has grown exponentially over the past decade, and shows no signs of slowing down.
By providing rapid, reliable translations, MT means businesses can maintain agility and meet the expectations of larger, more diverse global audiences. Consider the following use cases:
- Ecommerce: Frequent product listing updates can quickly overwhelm TMs, but require fast translation to support international sales. MT can handle these updates in near-real-time.
- User-generated content: Social media posts, forum entries, in-game chat, or support tickets often lack pre-existing translations in the TM, making MT an important tool to get an initial translation quickly.
- Rapidly evolving domains: In tech or scientific research, new terminology or rapidly changing content can outpace the growth of TMs. A custom-trained MT can learn updated vocabulary faster.
- Documentation with mixed content: Large user manuals with frequent revisions combine repeating content (great for TM) and new sections that benefit from MT. The synergy of both yields efficiency gains.
9. The best of both worlds
Machine Translation offers a number of critical advantages over Translation Memory alone, especially in handling new and large volumes of text efficiently, adapting to domain-specific needs, and continually improving over time.
While TMs remain indispensable for leveraging past, proven human translations, MT has progressed to a point where it can handle much of the heavy lifting—particularly when backed by advanced AI solutions like Phrase Language AI.
TMs and MT are not mutually exclusive but complementary. When integrated through an intelligent platform, you can harness the best of both approaches—reusing existing, validated translations while leveraging cutting-edge AI for unparalleled coverage, speed, and adaptability. The result is a workflow that is more efficient, consistent, and cost-effective than relying on either method alone.
Conclusion: Building smarter translation workflows
Machine Translation has moved far beyond its early limitations, becoming a strategic asset for companies managing growing volumes of global content. When thoughtfully combined with Translation Memories, glossaries, and automated quality checks, MT doesn’t just fill in the gaps—it helps teams move faster, scale smarter, and deliver consistent results.
At Phrase, we’ve built our platform to support this hybrid, AI-powered approach—blending your existing translation assets with advanced language technology to create faster, more flexible workflows. Whether you’re looking to reduce costs, accelerate time-to-market, or improve linguistic quality at scale, solutions like Phrase Language AI can help you get there.
Phrase Language AI
Learn how Phrase supports intelligent, secure, and scalable translation strategies,