Machine translation Phrase and beyond
98: Where Machine Beats Memory in Translation Speed
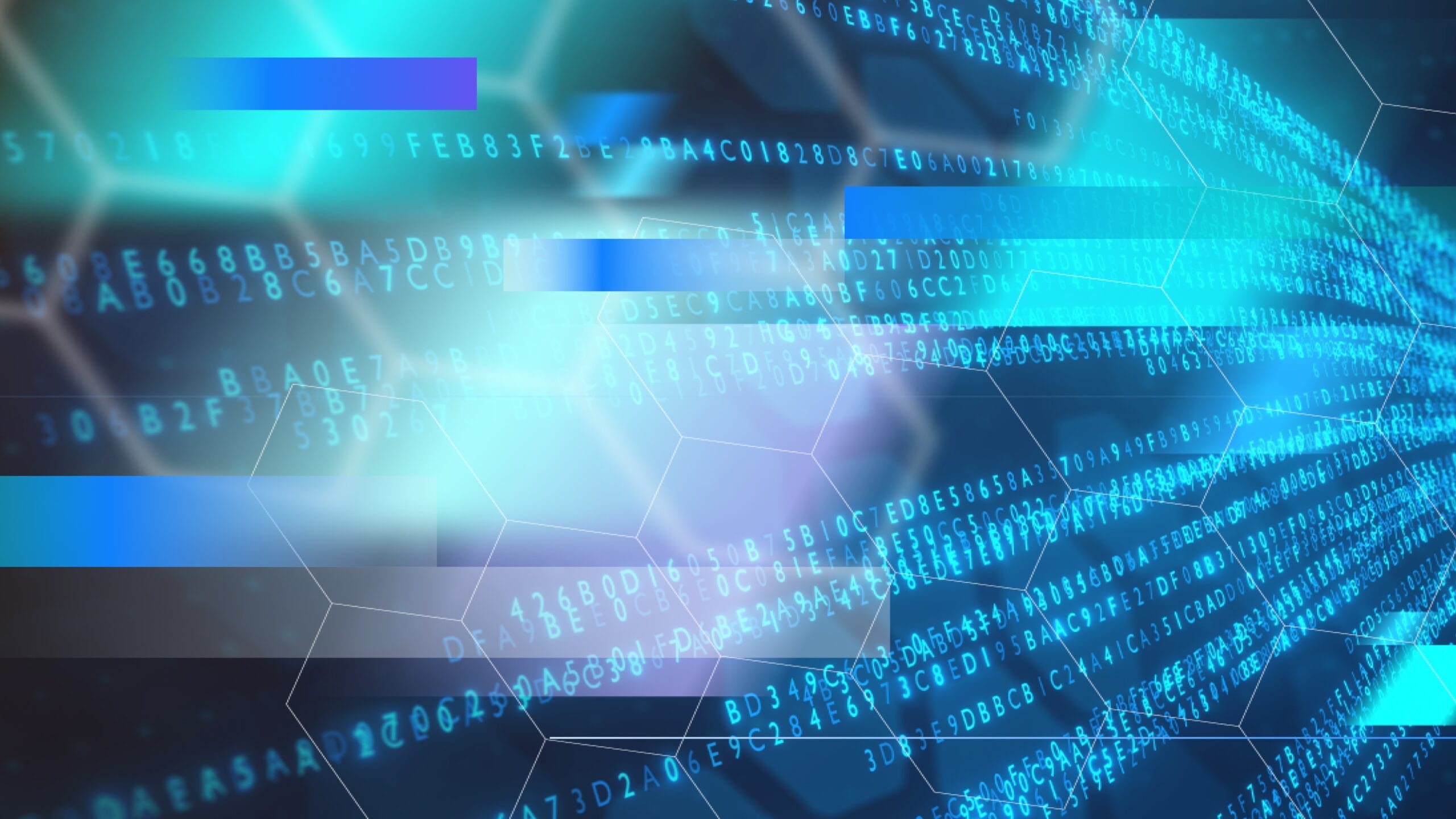
In our hyper-connected world, every second matters.
With AI driving the explosion of content, the speed at which information is delivered is crucial for staying competitive. Communicating in a language your audience understands is vital for reaching global markets—but how can we accelerate this process?
We decided to find out.
Crunching the numbers
We crunched a ton of data to figure out how customers can cut down on the time they spend translating content. 956,675,510 data points to be exact.
As you’d expect, segments with higher fuzzy match scores are quicker to edit. But once you hit about 93, things level out—below that, editing times don’t really get faster, since those TM segments tend to be pretty much rewritten from scratch.
Then, we compared editing times for Translation Memory (TM) segments and Machine Translation (MT) segments.
On average, editing MT-generated segments takes 5.8 seconds. The only TM segments that beat that are the ones with a score of 98 or higher.
So, unless you’re dealing with super-high fuzzy matches, machine translation is your best bet for saving time.
What exactly do we mean by “editing” time?
We actually track two times, thinking time and editing time. When a user clicks into a segment, both thinking and editing time begin to be tracked.
Once the user starts editing the segment, the counting of thinking time stops, but editing time continues to be recorded. When the user then clicks into a different segment, the counting of editing time stops.
When a user re-enters a previous segment, the counting resumes and any new times are added to the previous values.
If there is any editing upon re-entering, both thinking and editing times are updated. However, if re-entering a segment results in no editing, the times remain unchanged despite the segment being re-confirmed.
What about the coffee breaks?
There’s always that set of segments with unusually long editing times. These often happen when the linguist gets distracted mid-edit—maybe they’re checking their phone or getting a coffee.
Since the average editing time is around 20 seconds, we add a 50% buffer and remove any segments that take longer than 30 seconds. Sure, some longer edits might be legit, but most of the time, it’s just noise.
We tested whether cutting out these 30-second-plus segments affects the data.
On larger datasets, it barely makes a difference. But on smaller sets, it can be more noticeable.
This makes sense—if you take a “coffee break” in the middle of a small job, it’s going to have a pretty obvious impact on the total editing time.
That’s why we filter out those long segments to keep the data clean.
Why not just use edit distance?
The edit distance metric can be pretty misleading—it makes fixing a translation seem way easier than it actually is.
It simplifies everything down to just counting keystrokes for corrections, but completely ignores the brainpower and expertise required to make sure the translation is actually accurate.
What edit distance really misses is the thinking time. It’s not just about hitting the right keys.
Editing time, while not perfect, does a much better job of capturing that mental effort and complexity. It reflects the real work involved, not just the number of keystrokes.
Which segments made the cut?
To ensure an accurate view of job performance, we include all segments from completed workflows, even those with no editing time.
This helps to reflect the true impact of optimization. These are the segments we consider:
- We’re pulling in segments from jobs where all workflow steps are completed. We treat job completion as a sign that the segments are “done”.
- We include segments with 0 editing time, because if optimizing leads to more segments that don’t need any edits, that needs to be factored in.
Example:
Let’s say you have a 5-segment job, and originally 3 segments needed edits with times of 6, 8, and 10 seconds.
After optimizing the TM Threshold, now only two edits are needed—8 and 10 seconds.
If we only looked at edited segments, the average time would have gone from 8 seconds before to 9 seconds after, which wrongly suggests things got worse.
But when we include the untouched segments, the averages are 4.8 seconds before and 3.6 seconds after, which reflects the improvement.
For the same reason, we don’t exclude locked segments either.
Data for segments from shared jobs will only be visible to the organization that created the job (the buyer).
The curious case of the flat MT line
We’re correlating TM editing times with fuzzy scores, but for MT suggestions, fuzzy scores don’t exist—they’re not applicable.
Instead, we have QPS scores (Quality Performance Scores) for MT. Figuring out if there’s a link between fuzzy scores and QPS scores (so we can put them both on the same X-axis) is something we’ll explore in future research.
We’ll update you on that later. For now, we’re just using the overall average editing time for MT suggestions, which is why the line appears flat.
So, what does this mean for you?
The chart above shows averages across all data from all organizations. On October 2nd, we added a personalized dashboard to the TMS Phrase Analytics with data specific to your organization.
You are able to spot where the orange and purple lines intersect. This crossover point marks the golden TM threshold, helping you optimize for maximum effort savings.
Of course, different content types need different approaches.
Translating marketing content is a whole different ball game compared to technical documentation. The dashboard will also let you filter the data using predefined options to fine-tune the results to your needs.
Analytics, your Superpower for Localization Insights
Analytics is like the business world’s superhero, always swooping in to save the day by spotting where time and money are leaking.
We’re excited to take our Phrase Analytics to the next level with these new, actionable insights.
This is our first step into using ultra-granular, segment-level metadata. While this initial version might not be flawless, we’re committed to working closely with our customers to refine it and deliver tools that give them real oversight over their localization processes.
Stay tuned.
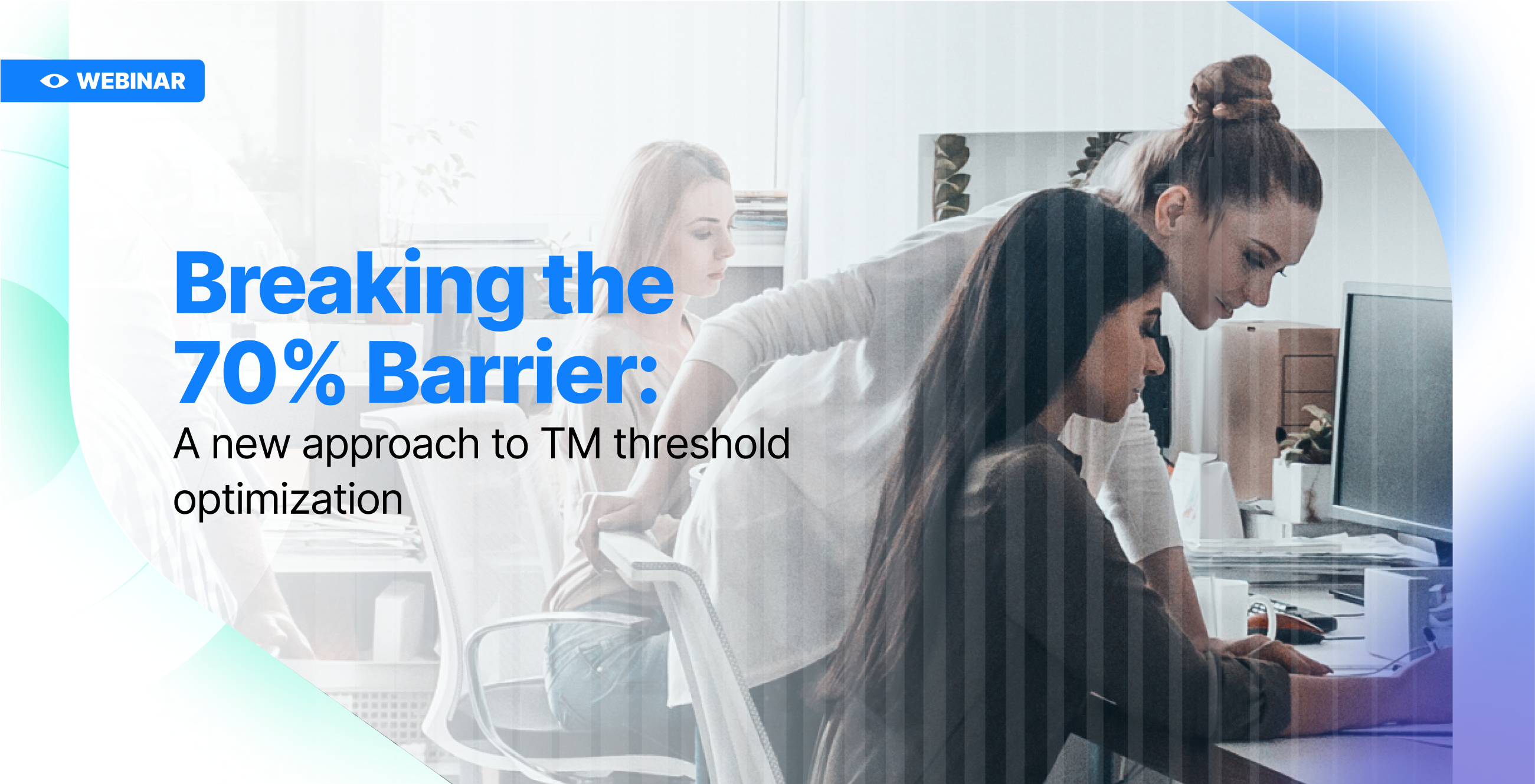
ON-DEMAND WEBINAR