Machine translation Phrase and beyond
Understanding Phrase Quality Performance Score (Phrase QPS) and Auto LQA: How they Unlock Hyperautomation on the Phrase Localization Platform
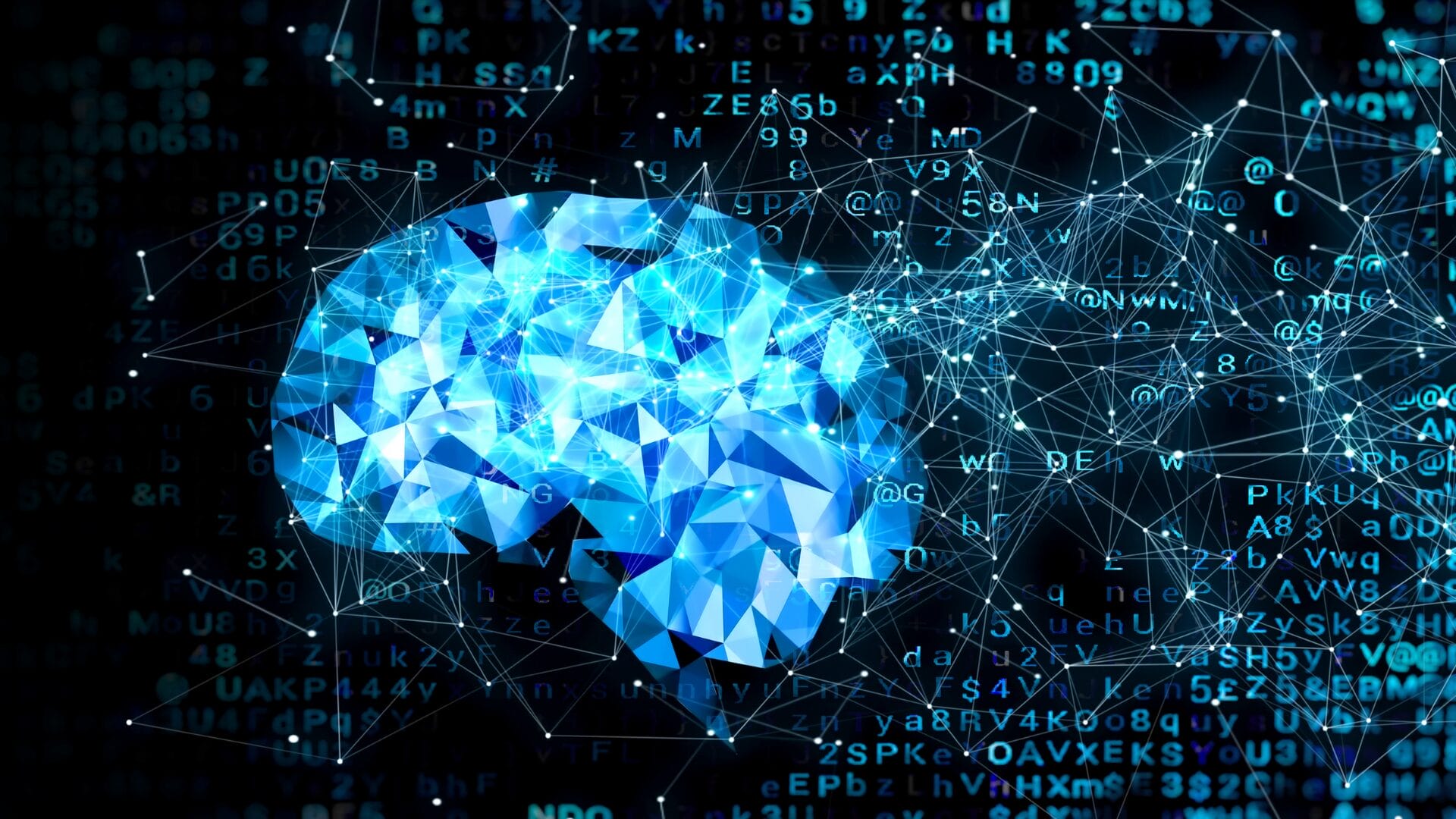
In this article, I focus on highlighting the rationale behind the development of our two Phrase-proprietary automated translation quality technologies: Phrase QPS and Auto LQA.
I explore how these two technologies, both built and based on a foundation of the MQM (Multidimensional Quality Metrics) framework, are designed to work in tandem to unlock hyperautomation capabilities for our enterprise customers.
Automated Translation Quality Visibility Unlocks Hyperautomation
The advances in neural machine translation (MT) and large language models (LLMs) in recent years, have been nothing but breathtaking.
For many of the major language-pairs and use-cases, state-of-the-art MT is now both accurate and fluent. However, despite such impressive strides, the use of MT alone still carries risks that are not tolerable for most enterprise applications.
Human translators are still required to perform post-editing and review – but this method is time-consuming and costly.
Alternatively, an organization may opt to employ MT without any human input at all. In doing so they take a gamble on possible embarrassing errors and misinterpretations that could be very damaging.
Enterprises seeking to streamline translation and localization workflows face a conundrum: how to adopt and accelerate automation without compromising quality or risking highly flawed translations. The Phrase Localization Platform already implements and offers workflows in support of both human post-editing of MT as well as full automation with MT.
However, we understand the need for more sophisticated workflows that allow our customers to maximize the value of MT while strictly optimizing for the right tradeoff balance between automation and level of quality risk. The solution lies in harnessing cutting-edge technology to provide unparalleled visibility into translation quality at scale.
The goal is to enable organizations to automatically detect and address low-quality translations efficiently within the translation workflow process, minimizing the need for extensive human intervention. Phrase QPS was designed specifically to address this need.
Phrase QPS assigns quality scores at the segment level, which are then aggregated to the document and job level. Workflow “Gating” decision-points are then implemented to support two major complementary decisions:
- At the job-level: is a translated job of sufficient quality to be completed without further human editing or review?
- At the segment-level, for jobs that are sent to human editing: which segments are of sufficiently high quality and can be “blocked” from human editing and correction?
Crucially, Phrase QPS is designed to operate seamlessly across diverse translation scenarios.
This not only includes machine-translated content but also human-edited MT and traditional human translation. This versatility ensures that enterprises can maintain rigorous quality standards across all of their localization workflows, regardless of the translation method employed.
Phrase QPS, Auto LQA and MQM… and How they work in Tandem
The most reliable process for assessing the quality of translations has long been a human-expert-intensive process known as Linguistic Quality Assessment (LQA). Human LQA has evolved, with increasing adherence in recent years to the Multidimensional Quality Metrics (MQM) framework. This approach has been further consolidated by the recent release of ISO-5060, an ISO standard based on MQM.
MQM is a comprehensive framework for assessing translation quality over several dimensions. It takes into account different quality requirements such as fluency, adequacy and adherence to terminology and categorizes them into error types. This offers users a structured way of evaluating the accuracy and quality of translated content.
Beyond its value in providing consistent and structured MQM evaluation feedback, MQM enables improvements to the various stages of translation leading to consistency and dependability in translation quality assessment. Its multidimensional nature allows for a nuanced understanding of translation performance.
This makes it a useful tool for translators, researchers and stakeholders involved in the localization and language industry.
The execution of human LQA for translation using the MQM framework typically involves trained linguists or evaluators who systematically go though translated content based on the MQM predefined quality criteria. Evaluators compare the target text with its source in order to identify markup and categorize translation errors and discrepancies, along with their severity.
Consequently, an overall score for the entire translation job is calculated based on a severity-weighted scoring mechanism. This aggregates the various issues identified during evaluation and annotation of the translated segments leading to an overall MQM score. Employing LQA within frameworks offered by MQM enables a rigorous examination of translation quality that can inform decisions made by stakeholders geared towards improving their localization process.
Due to its cost and speed limitations, Human LQA has long been restricted to offline quality assessments on small samples of content. Yet many enterprises allocate major portions of their localization budgets to LQA.
With the recent advent of LLMs, full automation of Human LQA, at impressive levels of accuracy, has now become possible.
For this purpose, we developed Auto LQA.
This LLM capability is fully-automated and is orders of magnitude faster and less-costly than Human LQA.
It can be used for use-cases where automated analysis is deemed sufficient, as well as an automated “pre-annotator” for Human LQA (similar to the way MT is used in conjunction with human MT post-editing).
Once Auto LQA annotates all segments in a job, an MQM score can be algorithmically calculated. This can be done in exactly the same way as is typically done with human LQA.
So if we now have Auto LQA, why do we still need Phrase QPS?
In an ideal world, one AI component would indeed be capable of doing both, and we expect that to emerge in the not-too-distant future.
While Auto LQA represents a significant leap forward, its utility is currently still hindered by the relatively slower speed and higher cost of LLMs, limiting its scalability. QPS addresses this challenge by training a smaller, faster and less costly AI model.
This model predicts the quality score that would be assigned by an MQM annotator (or by Auto LQA), without generating the detailed annotation. While slightly less accurate than its human and Auto LQA counterparts, QPS meets the crucial requirements of speed and cost-effectiveness.
Phrase QPS is trained on Human LQA MQM annotations supplemented by synthetic data generated through Auto LQA, and then refined by human corrected iterations.
This ensures that Phrase QPS balances precision and scalability. Furthermore, the fact that QPS and Auto LQA are separate and independent AI models presents an opportunity for mutual validation. This enhances the overall reliability of assessment and validation of both models (More about that in a future article!).
The Bottom Line
To sum up, the field of estimating translation quality is undergoing significant changes due to technological advances and methodological improvements. Auto LQA and Phrase QPS represent two innovative pathways toward achieving automation in evaluation, each with their own set of compromises.
Phrase QPS can scale translation quality visibility by unlocking new levels of translation hyperautomation at measurable quality risks.
Auto LQA significantly reduces LQA costs while simultaneously generating valuable data required for training an accurate Phrase QPS model.
New processes of assessment involving both human and automated judgments can help achieve reliable and scalable TQA (Translation Quality Assessment) that lead to streamline localization processes and effective multilingual communication.
This innovation signifies a critical turning point in the market, revolutionizing automation and scalability within localization. It lays the groundwork for hyperautomation, where content is seamlessly processed through various AI and machine learning techniques and workflows. This will transform both the efficiency and precision of multilingual content creation—a vital component for managing the growing volume of content in today’s globally connected world.