Machine translation
Phrase Quality Performance Score: What You Need to Know
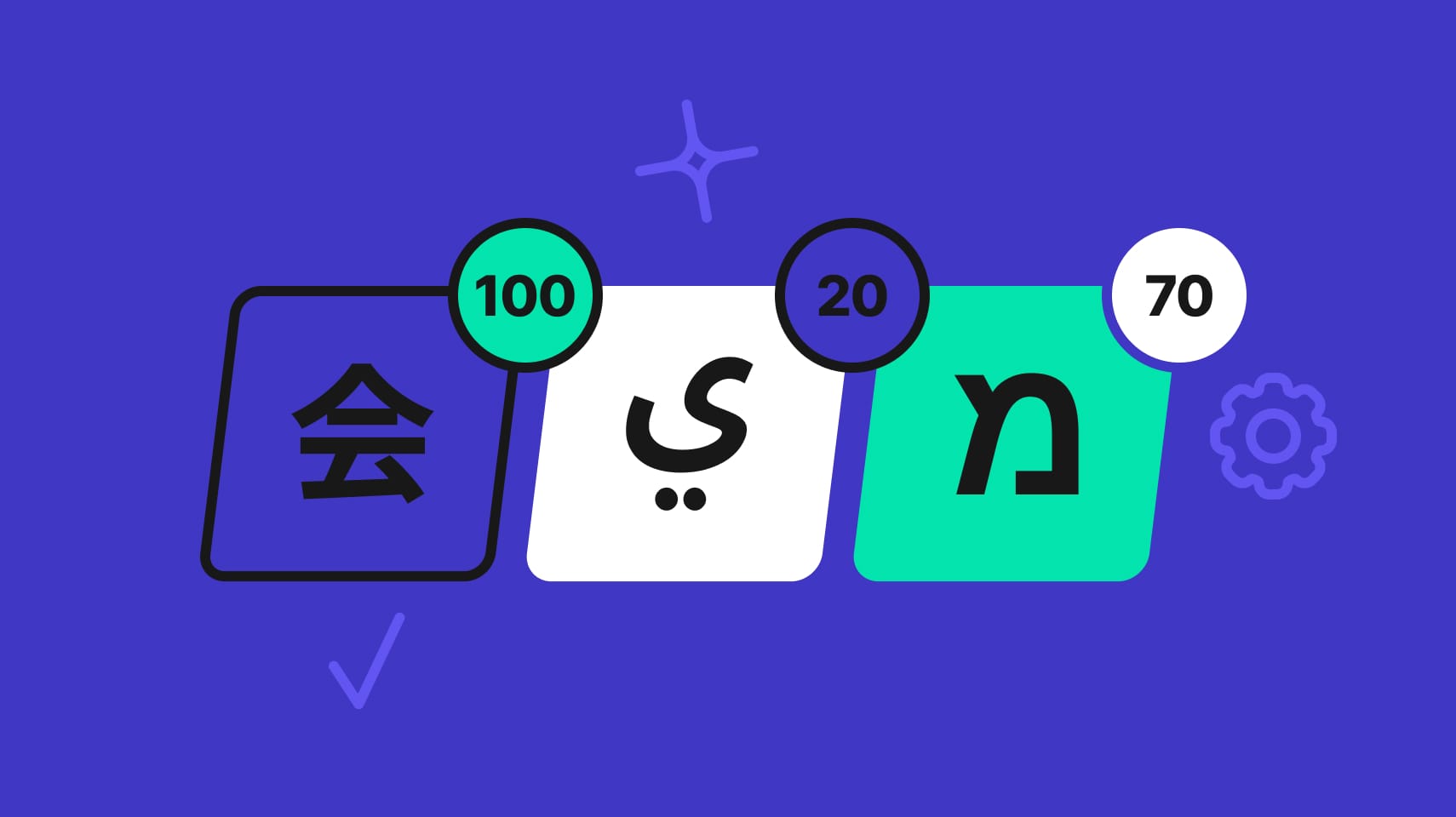
Translation quality is a critical factor for global enterprise customers striving to communicate effectively on a global scale. Phrase has been a trailblazer in automated translation quality evaluation, commercially releasing one of the first quality estimation algorithms back in 2018. Since then, it has provided insights on translation quality to thousands of customers, evaluating billions of segments.
Now, we present the next evolution in quality evaluation: Phrase Quality Performance Score (Phrase QPS), designed to integrate seamlessly into the Phrase Suite and enable hyper-automated and scalable translation workflows. By making it possible to optimize the cost and time associated with localization, we empower enterprise customers to expand their reach.
What is Phrase QPS?
Phrase QPS is an AI-driven system that predicts quality scores based on the Multidimensional Quality Metrics (MQM) framework. Capable of providing instant quality insights for both human and machine translation, Phrase QPS scores range from 0 to 100. Unlike its predecessor, MTQE, Phrase QPS relies on a combination of human post-editing data and structured evaluation in line with the industry-standard MQM framework. Notably, Phrase QPS goes beyond segment-level scores, offering document-level assessments—particularly advantageous for large-scale machine translation use.
Why use the MQM Framework?
The MQM framework is renowned for its robustness in evaluating translation quality and is widely adopted in the industry as the gold standard for translation review. MQM is already utilized in the Phrase TMS’s Linguistic Quality Analysis (LQA) feature, which allows for the identification and categorization of translation errors according to type and severity. MQM’s ability to summarize errors at a segment or document level through predefined calculations adds a layer of grounding and granularity to translation quality assessment.
How is Phrase QPS integrated into the Phrase Suite?
Phrase Strings
With the release of Phrase QPS, automated quality scores are finally available for users of Phrase Strings. Translators working in the editor will see AI-generated quality scores from 0 to 100. Scores are automatically assigned for both human-edited and machine translation, making quality assessment real-time. Not a fan of automated scores? Then you can toggle them off in the app settings.
Phrase TMS
Phrase QPS builds on and surpasses Phrase’s previous MT Quality Estimation and adds some new management features. Quality scores are provided in all the same places as MTQE and are used exclusively for machine translation. Unlike MTQE, which grouped scores into four categories, Phrase QPS provides granular scoring from 0 to 100. Phrase QPS not only helps translators quickly review content directly in the editor but can also be used to automate workflows further. Project managers now have the option to set custom thresholds, automatically confirming segments that exceed specified scores to avoid unnecessary content review.
Phrase Language AI via API
Phrase QPS doesn’t just provide scores for strings and segments. Phrase Language AI via API can provide document-level scores swiftly through the API. This enables real-time identification of poor-quality translations, ensuring a streamlined quality control process for machine translation at scale.
What does Phrase QPS mean for the future of the Phrase Suite?
Phrase QPS marks a significant leap forward for the optimization of speed, cost, and accuracy of translation workflows within the Phrase Suite. Users of Phrase Strings, Phrase TMS, and Phrase Language AI via API have Phrase QPS immediately at hand as a versatile tool for achieving high-quality translation at scale.
Quality scoring at the segment and document level is the key to automating and scaling localization workflows. By unlocking insights into quality, smart workflows can route the right content to the right actions. Phrase QPS is part of a wider vision we have for AI and quality assessment in the Phrase Suite. We are looking forward to sharing some further innovations with you in the near future.
We encourage users to try out Phrase QPS, and unlock the potential for a whole new standard in translation excellence through the Phrase Suite.
Speak with an expert
Want to learn how our solutions can help you unlock global opportunity? We’d be happy to show you around the Phrase Localization Platform and answer any questions you may have.