Translation management
Time to Rethink How Much We Depend on That “70% TM Threshold” Rule
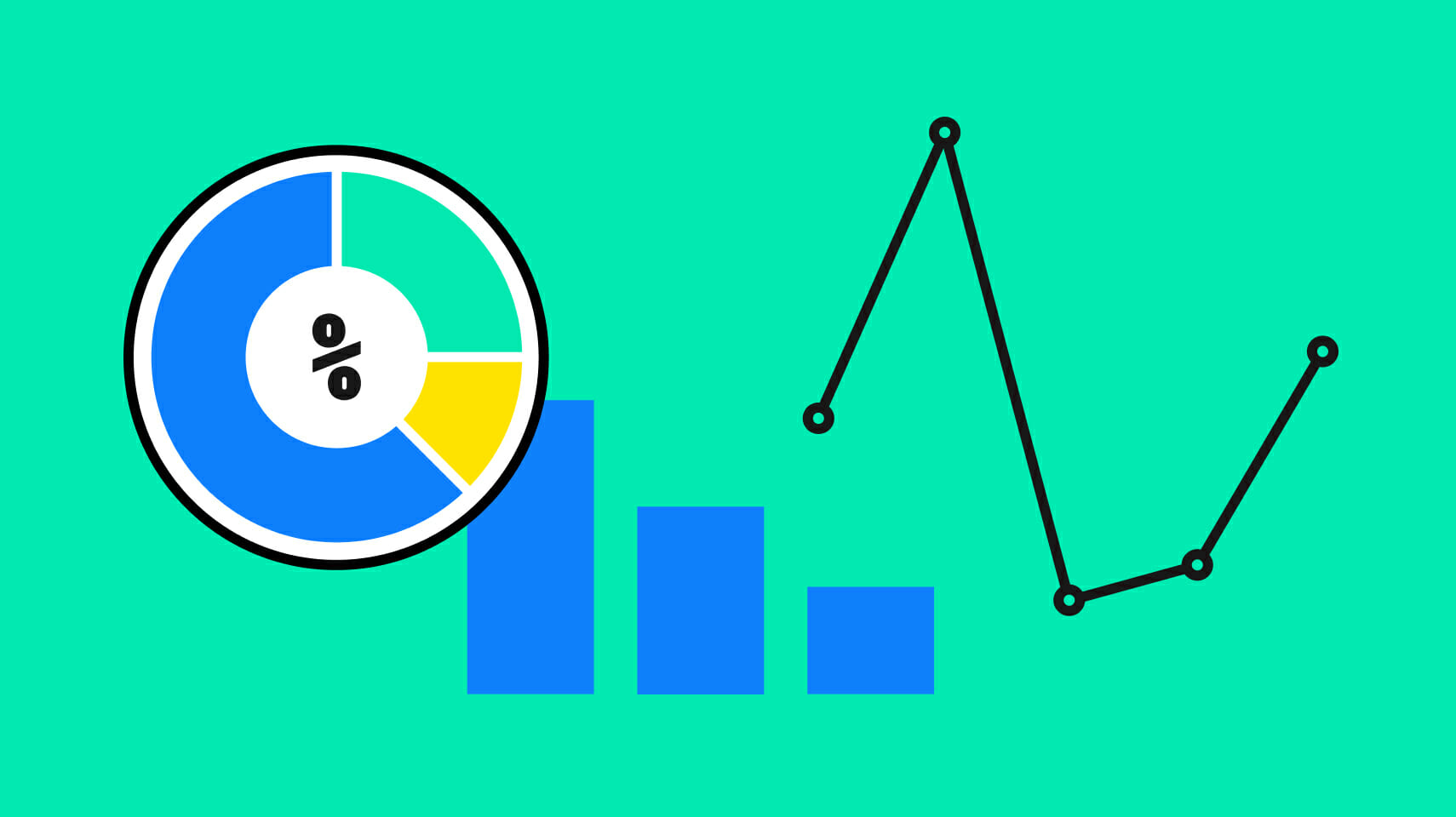
In my previous post, we looked into how optimization through analytics can boost our translation productivity. We highlighted two key findings: the need to tweak our suboptimal TM Threshold settings and an eye-opening insight that improving the quality of translations produced by the TM pre-translation by just 1% can cut down segment editing time by a whole 3 seconds. Today, we’ll pick up right where we left off and dive into the blind chart we teased at the end of our last post.
Today, with labels:
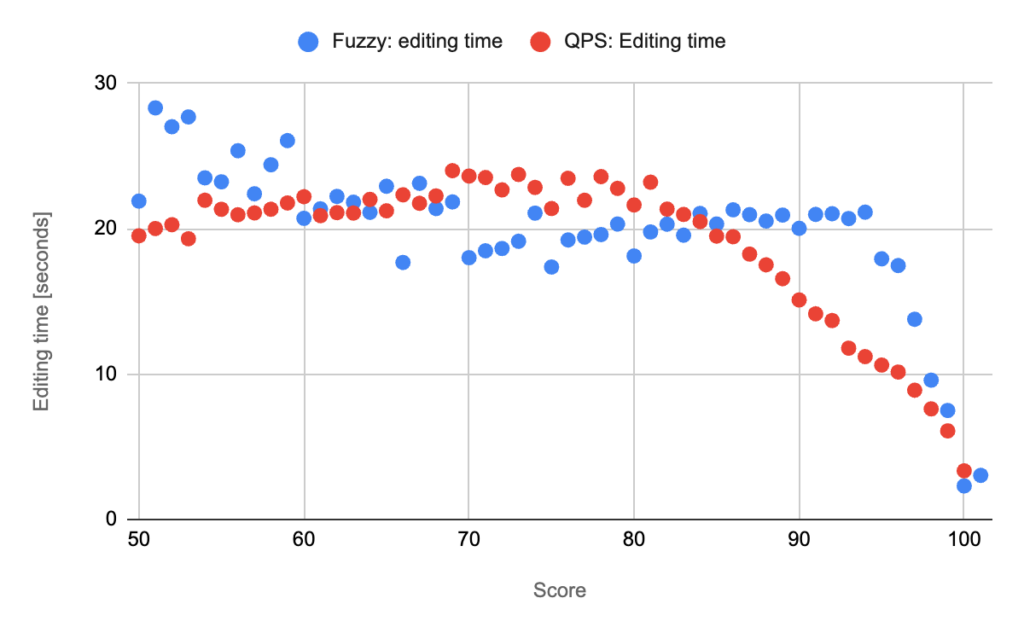
Before we dive into interpreting the chart, it’s crucial to understand the distinct nature of the two scores. Let’s first break down what these scores really mean, how they interact, and why they are critical for our optimization strategies. By doing so, we can better appreciate the insights the chart offers and make informed decisions to enhance our translation workflows.
TM fuzzy matching is the helpful elf in Santa’s workshop. It scours through the TM, spotting a source segment that’s kind of like what you’ve already translated, and goes, “Hey, this is the closest you’ve translated so far.” But unless that score hits a perfect 100 or a magical 101, it’s not the exact match, and it mostly likely needs a linguist’s touch to polish it up. So, while TM fuzzy matching is super helpful—it’s always just Santa’s helper. For the world of automation, it means you can’t automate with TMs only.
Machine Translation (MT) is like aiming to be the big guy in red himself, striving to produce the final translation on its own. It has “historical matches” in its training set, where they’re essentially baked into the neural network through machine learning. This brainy network can think outside the box, allowing it to translate new texts it hasn’t encountered before. And that’s the big difference from TMs that can’t.
TM Fuzzy matching is essentially a simple compare algorithm, which can’t produce final translation. But because we know precisely how it functions, we can confidently rely on the Fuzzy scores it generates. TM’s ability to deterministically produce a score we trust stems from its straightforward, predictable n-gram algorithm.
MT has the capability to produce a final translation, which is pretty impressive. However, the catch is that we don’t quite know how it came up with its results. The neural networks behind MT operate in ways that can sometimes feel like a black box, making it a bit of a mystery.
That’s exactly why so much effort and resources have been devoted to developing a reliable algorithm to score MT outputs. At Phrase, this has been a pivotal research area for many years. The algorithm has evolved significantly over time, most notably replacing the original MTQE, which focused on estimating the edit distance needed to correct MT output. It has since shifted to Phrase Quality Performance Score (QPS), which incorporates human post-editing data and structured evaluations aligned with the industry-standard MQM framework.
Essentially, the Phrase QPS is designed to mimic what you’d expect if a linguist were scoring translations based on the MQM framework. This means the score reflects a comprehensive, human-like assessment of translation quality, evaluating it with the same rigor and criteria a professional linguist would use. That’s the type of data we train it on.
How do we know the scores from Phrase QPS are reliable? This is where the chart we mentioned earlier comes into play. It demonstrates a solid correlation between the QPS scores of a segment and its editing time. It shows that higher scores require less intervention from linguists. Essentially, the better the score, the less the need for costly and time-consuming revisions. This kind of correlation is exactly what we want to see—it confirms that the scoring algorithm is doing its job effectively. And despite being a NKOTB, it has its place as a trusted partner in our automation toolkit.
Looking further at the data, we see something pretty interesting: TM Fuzzy scores actually only beat out MT when the matches are perfect 100s or 101s. This really throws a wrench into that whole “70% TM Threshold is industry standard” thing we’ve been sticking to. It looks like that rule might not be holding up unless the matches are nearly perfect, which definitely puts that standard in a new, less flattering light. Maybe it’s time to rethink how much we depend on that 70% rule.
If you’ve already ditched the 70% TM Threshold rule, then you might be ahead of the curve. But in my last post, I highlighted how 70% remains the most commonly used threshold. This established norm has a hefty price tag associated with it.
Another point to note is that regardless of whether the initial translation was done by TM or MT, a segment that was poorly pre-translated typically requires about 20 to 25 seconds to fix.
Lastly, it’s important to note the steepness of the red line, which indicates a decrease of about 1 second of editing time per segment for every 1-point reduction in the QPS Threshold in TMS pre-translation. This suggests that slightly lowering the quality threshold could lead to significant time savings.
How much could your organization save? Get ready for your journey to uncover the hidden treasures of cost-saving secrets! Discover the optimal TM and QPS Threshold values for your projects. The answers to these pivotal questions are just around the corner.
Join our expert-led webinar
October 16, 2024 at 4:30 pm CEST | 10:30 am EDT
Join us for a game-changing webinar where we challenge the industry’s reliance on the 70% Translation Memory (TM) threshold for pre-translations. We’ll reveal how leveraging machine translation for TM scores between 70-98% can boost translation productivity and speed up project timelines.