Machine translation
Creating an Effective Machine Translation Strategy in 10 Key Steps
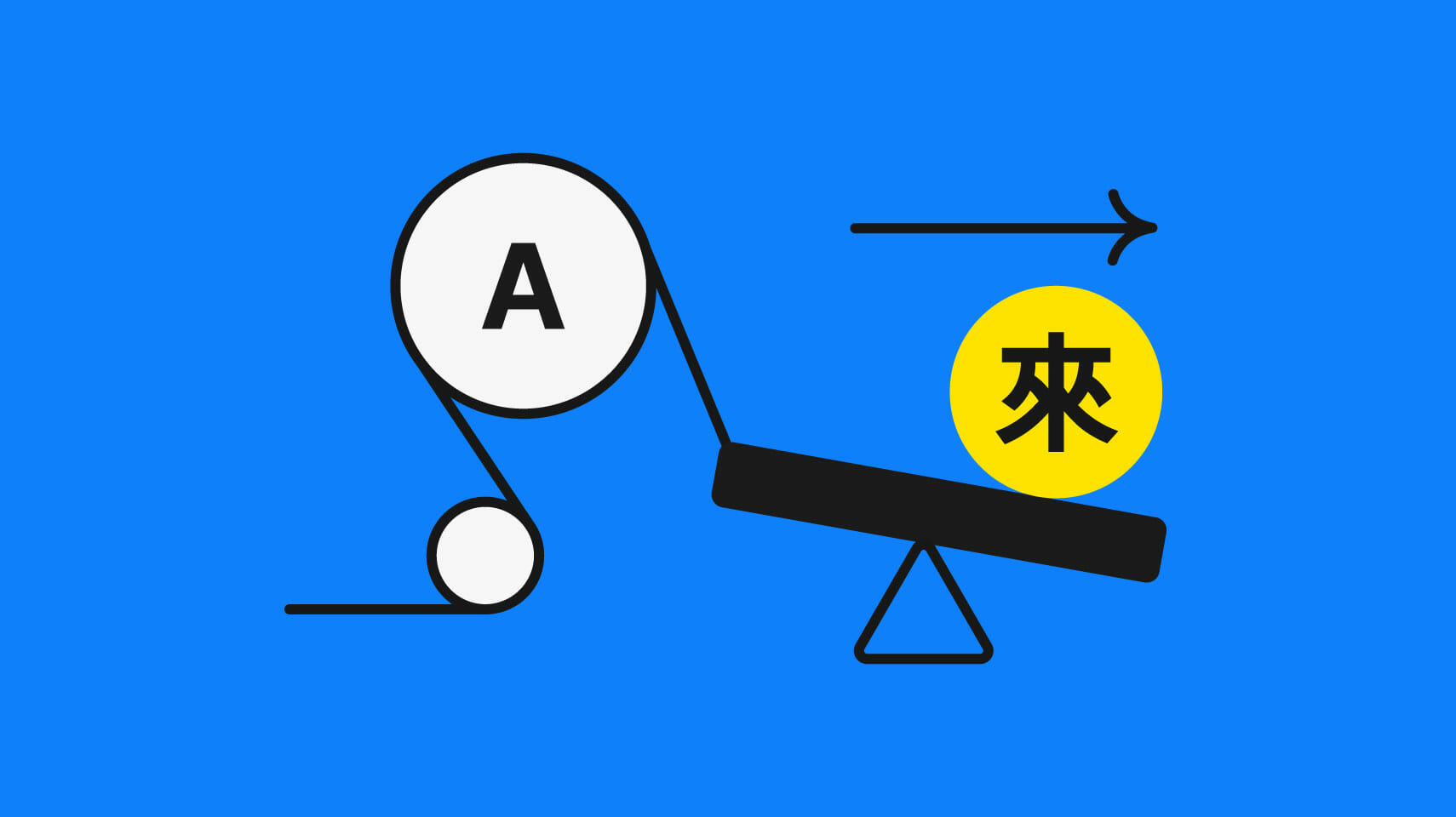
Costly mistakes in global business often happen because of insufficient planning. Missed opportunities caused by a lack of automation powered by artificial intelligence and lost revenue resulting from inefficient processes are common pitfalls that can be easily avoided when a company has a clear understanding of the necessary actions to take.
Machine translation (MT) is no exception to this rule. While it’s increasingly becoming part of localization strategies, many growing businesses still lack a well-defined approach to it. To develop a robust machine translation strategy that optimizes globalization efforts, it’s crucial to consider the following 10 steps.
Why is a machine translation strategy important?
Most large enterprises around the world have moved beyond the why of using machine translation for global growth. The rise of neural MT, in particular, prompted companies to see MT as a viable productivity tool for entering new markets more quickly while keeping costs low.
From theory to practice, though, not everything is so straightforward—many companies get overwhelmed with adopting a method that fits their goals and business needs. From what type of content to run through machine translation to how to use machine translation post-editing (MTPE) effectively and at what price, there are many variables to consider.
A machine translation strategy is important to answer these questions—and more—while helping you:
- Save time and money: Machine translation helps you localize content faster and more affordably—so long as you use it strategically.
- Get it right the first time: You avoid wasting time and effort by ensuring you’re using the right machine translation engine for your goals, in the right use cases, and with the right processes in place.
- Ensure fully localized content: Well-directed machine translation efforts mean no content is left behind—so your user and customer experience don’t suffer. It’s just a matter of prioritizing what assets to use human translation for, which ones to post-edit, and which ones to run through MT as-is.
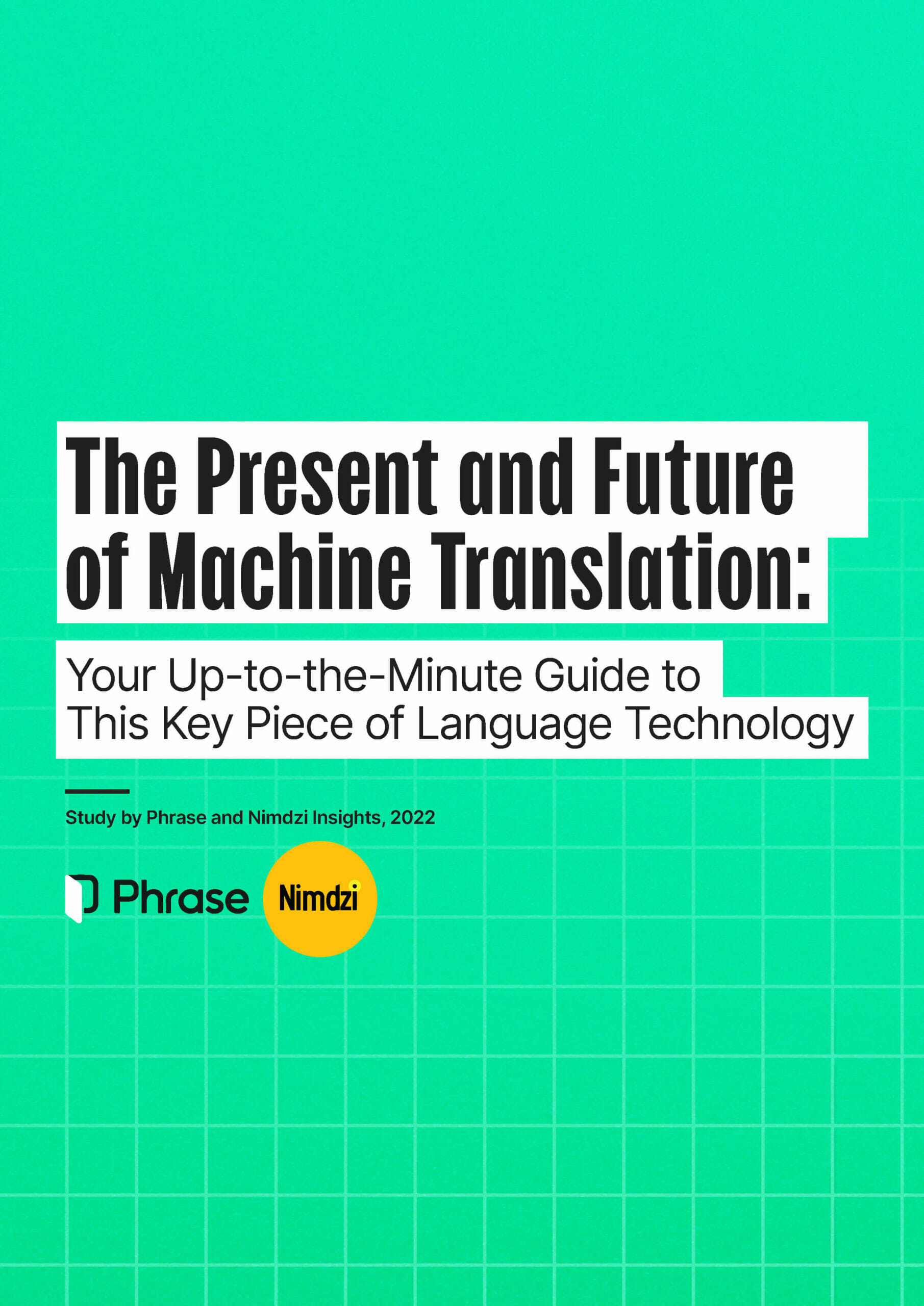
Download for free
Your up-to-the-minute guide to machine translation
Learn about new technologies to improve machine translation output quality, the latest on MT post-editing pricing models, and how to best shop for machine translation.
10 steps to creating a winning machine translation strategy
Before you can fully make efficient and effective use of machine translation, you need to map out a plan that works for you. Here are the 10 steps to help you do this.
Step 1: Define your goals
Determine what you hope to accomplish by employing machine translation as part of your localization program. Are you looking to save on costs? Increase the time to market? Localize content that would otherwise not be prioritized due to lack of resources?
Machine translation can offer all of these benefits, but it’s essential to know exactly what your goals are. For example, if cost savings are your primary objective, you can work out ways to leverage raw (i.e., unedited) MT as much as possible according to your use cases. You can then prioritize post-editing and human translation only when absolutely necessary (e.g., for win-back email campaigns or other content where errors could prevent you from reaching your goals).
Step 2: Analyze your content
Not all content is created equal, and not all assets are equally important for your strategic goals. Internal documentation isn’t the same as customer-facing content, your home page will have higher visibility than some other pages deep down in your site’s architecture, and some content will perish more quickly than others (e.g., product reviews).
To apply machine translation efforts where they’ll bring the most benefits without compromising quality, consider classifying the content that you need to translate in terms of:
- Visibility (high, medium, or low)
- Audience (internal vs. external stakeholders and customers)
- Importance to your goals (critical or not critical)
- Perishability (permanent or temporary)
The rule of thumb is that any content permanent, highly visible content targeted at external stakeholders should involve a human translator to some degree. This could take the form of either full post-editing (more on full vs light post-editing below) or from-the-get-go human translation.
Step 3: Determine your language pairs
Different machine translation engines on the market are more suitable for certain language pairs than others. This has to do with how much data they have available to learn from. To train a neural MT engine, you need millions of examples—machine translation can only get better if the engine has a lot of data to study. Some regions, like Asia, are in the early stages of MT adoption, and many engines have yet to train for some Asian languages, despite Chinese and Japanese providing a great amount of data already.
Moreover, some language combinations and directions tend to produce better results than others. Some studies report language pairs involving Romance languages—Spanish, French, Italian, Portuguese, etc.—as producing the best results.
Step 4: Create a plan and budget
How much money and time you can spend on machine translation will determine how much you can accomplish. Create a timeline with realistic goals based on your available resources, set a budget for third-party costs, and decide how much you can afford to pay for human post-editing, 100% human translation, or transcreation services.
For example, say you have an upcoming product launch and enough budget to invest in full human translation, but the timeline is tight. You may opt to run anything that’s not too visible through an MTPE workflow, and have your team of translators work manually on marketing materials and product pages that are highly visible and potentially more critical for your strategy.
Step 5: Choose the right machine translation provider
Once you have a clear overview of your needs and goals, you’re ready to choose the MT technology that best fits your use cases. Choosing a machine translation solution that best meets your specific needs and budget isn’t always easy, and it can involve a lot of trial and error.
When evaluating machine translation technology vendors, consider:
- Feedback from your translators: You can run a blind test with different machine translation engines and ask your translators to evaluate the quality of each engine’s output.
- Research by industry thought leaders: Look at studies or reports published by recognized industry experts that compare different engines in terms of quality, speed, and cost. Compare their insights with your translators’ evaluation.
- Automated scoring methods: Several automated scoring methods such as BLEU, TER, or chrf3 can help you gauge quality.
- Fully automated assessment: Modern, cloud-based translation management systems come with built-in quality analysis tools that use AI to assess the best-performing MT engine for each of your projects, depending on the content type and language pair.
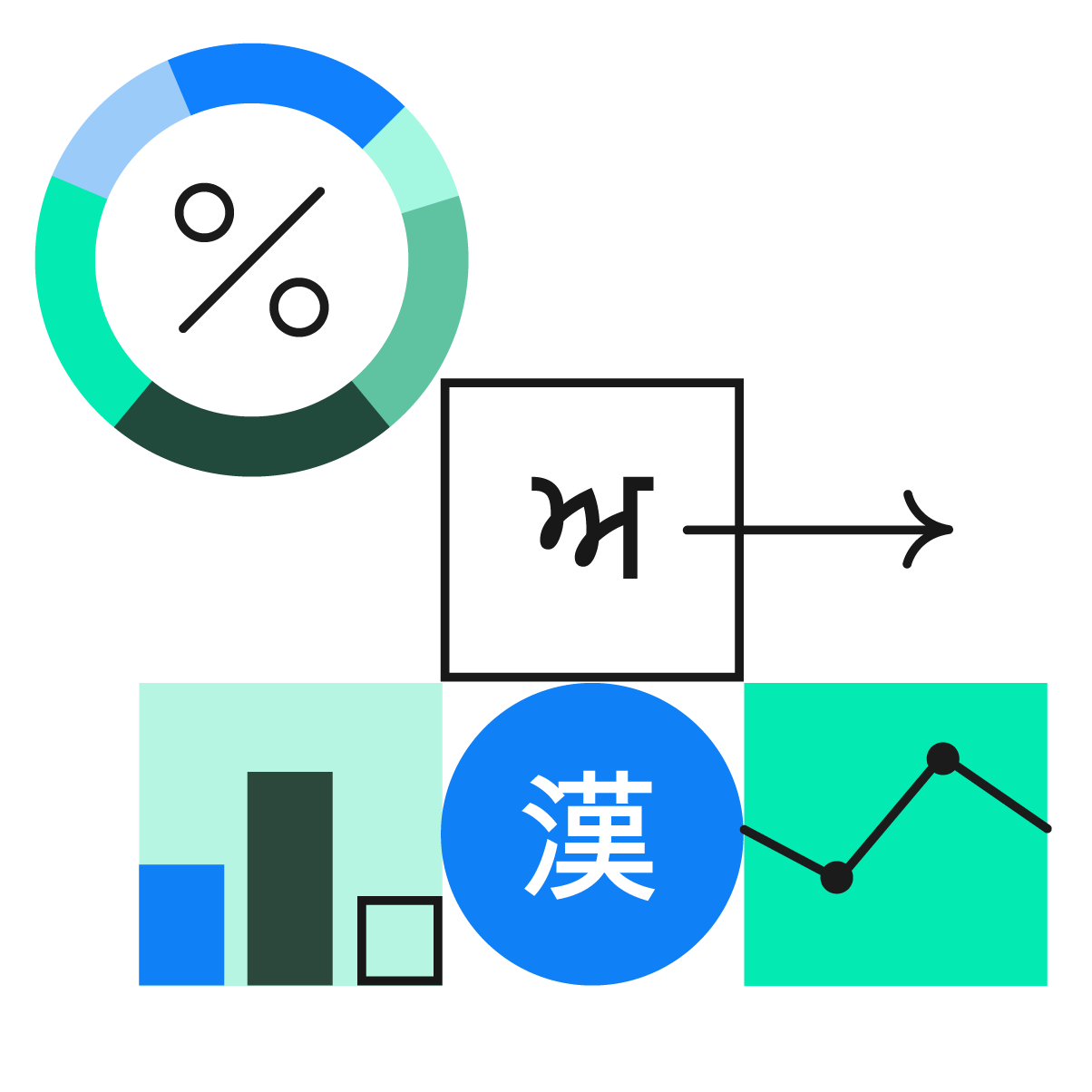
Interactive MT report: Uncover top performers
Find out how leading machine translation engines perform for different content types using the latest data in our quarterly machine translation report.
Step 6: Check your MT provider’s privacy policy
Be it Google Translate or Amazon Translate, when you’re entrusting a third party with your sensitive data, such as customer information or product specifications, it’s essential to make sure they abide by the most rigorous security standards. Most public MT providers, for example, offer translations in exchange for your data—which they use to train their AI and improve their services.
As an example, see below how DeepL’s data privacy policy differs between their free and paid services:
- Free service: “When using our translation service, please only enter texts that you wish to transfer to our servers. We process your texts, the documents you upload, and their translations for a limited period of time to train and improve our neural networks and translation algorithms.”
- Paid service: “After complete performance of the contractually agreed services all submitted texts or documents and their translations will be deleted. When using DeepL Pro, your texts will not be used to improve the quality of our services.”
Most providers apply similar rules, so make sure you read them carefully and check with your legal department if needed.
Step 7: Train your machine translation engine with your own data
The more data you provide to the MT engine, the better it will become over time. Unlike generic MT engines such as Google Translate, DeepL, or Amazon Translate, custom MT engines let you feed your own domain-specific data into the engine to train it for better results. If you translate a lot of the same content, training your engine with your own data will save you time and money, and increase the accuracy of the MT output.
Consider that custom MT engines tend to be slightly more expensive than generic ones, require more management and periodic maintenance, and that you might need to invest time and resources in curating training data.
Step 8: Consider machine translation post-editing
As we’ve seen, post-editing (PE) is an often necessary part of the machine translation process. It involves having human translators correct and review the output of an MT engine while keeping some of its suggested translations. This hybrid workflow aims to reduce costs while still ensuring quality.
The level of post-editing depends on the type of text and target audience. The two main types of PE are:
- Light post-editing (LPE): Post-editors just have to correct the raw MT output only where absolutely necessary, keeping as much of the MT output as possible while ensuring high quality. The focus is on the bare essentials of accuracy and legibility.
- Full post-editing (FPE): In this case, post-editors seek full accuracy, fluency, and naturalness in the output. Stylistic and tonal elements as well as cultural adjustments are just as important as grammatical and terminological accuracy.
It can be useful to think of LPE and FPE as being on a spectrum rather than a binary choice. Make sure to follow best practices when post-editing machine translation output, and consider selectively prioritizing content segments of higher business value for deeper post-editing.
Step 9: Agree upfront on a pricing model
Deciding on a pricing model for your MT projects is pretty straightforward: Most standalone solutions use a pay-as-you-go model for characters used. Alternatively, if you plan to use MT as part of a translation management system—for example, through Phrase Language AI, the MT add-on for Phrase TMS—subscription-based pricing is more typical. With Phrase Language AI, for instance, your subscription includes unlimited MT for post-editing.
The more complex decision is how to pay post-editors—it often doesn’t make sense to pay them the same as for pure human translation, but the quality of the MT output can really vary. The level of post-editing required can go from no changes to a comprehensive rewriting.
Because of this, there are 3 popular models for compensating post-editors:
- Effort-based pricing: Your vendor charges you proportionally to the amount of effort required for post-editing. This could be an hourly or per-word rate, established upon completing the project based on how much editing it needed. The main disadvantage of this model for large enterprises is that it can be hard to budget and manage expenses.
- Traditional per-word pricing: This model is much easier to forecast, as you agree on the per-word rate upfront and it applies no matter how easy or difficult a text may be to post-edit. However, for companies processing a lot of data in continuous workflows, the breakdown-by-project that per-word pricing often entails can be a bit cumbersome.
- Hybrid pricing: This model combines both effort and per-word pricing. For example, a vendor could apply an estimate at or near what a fully manual translation would cost, then discount it if the job was easy to post-edit. Another approach is to charge an hourly rate but with a cap, so that the total cost never exceeds a fully manual translation would cost based on ordinary per-word rates. In either case, the final cost of the project cannot exceed the initially quoted amount.
Step 10: Deploy, monitor, and measure results for continuous improvement
It’s also important to monitor MT output over time and re-evaluate it periodically to identify any changes that might require adaptations or improvements. This can include changing engine settings, updating training data, identifying new use cases, and if necessary, changing processes.
Track metrics such as translation accuracy and response time to ensure you’re getting the best possible results. Finally, make sure your machine translation strategy is constantly evolving—consider if the technology, processes, and resources you need might change over time.
Think big, act strategically, and don’t rush in
These 10 key steps are the foundation of a comprehensive machine translation strategy and will help you avoid costly mistakes in your globalization initiative. Take your time to assess the scope of your project and the resources you have available, and map out your strategy step-by-step.
By following these steps, and with proper planning, knowledge, and resources, you can open your doors to new markets cost-effectively without risking quality or customer satisfaction.
Unlock the power of machine translation
Discover advanced machine translation management features within our enterprise-ready TMS and create new business opportunities worldwide more quickly and efficiently.