Machine translation
How Accurate Is Google Translate?
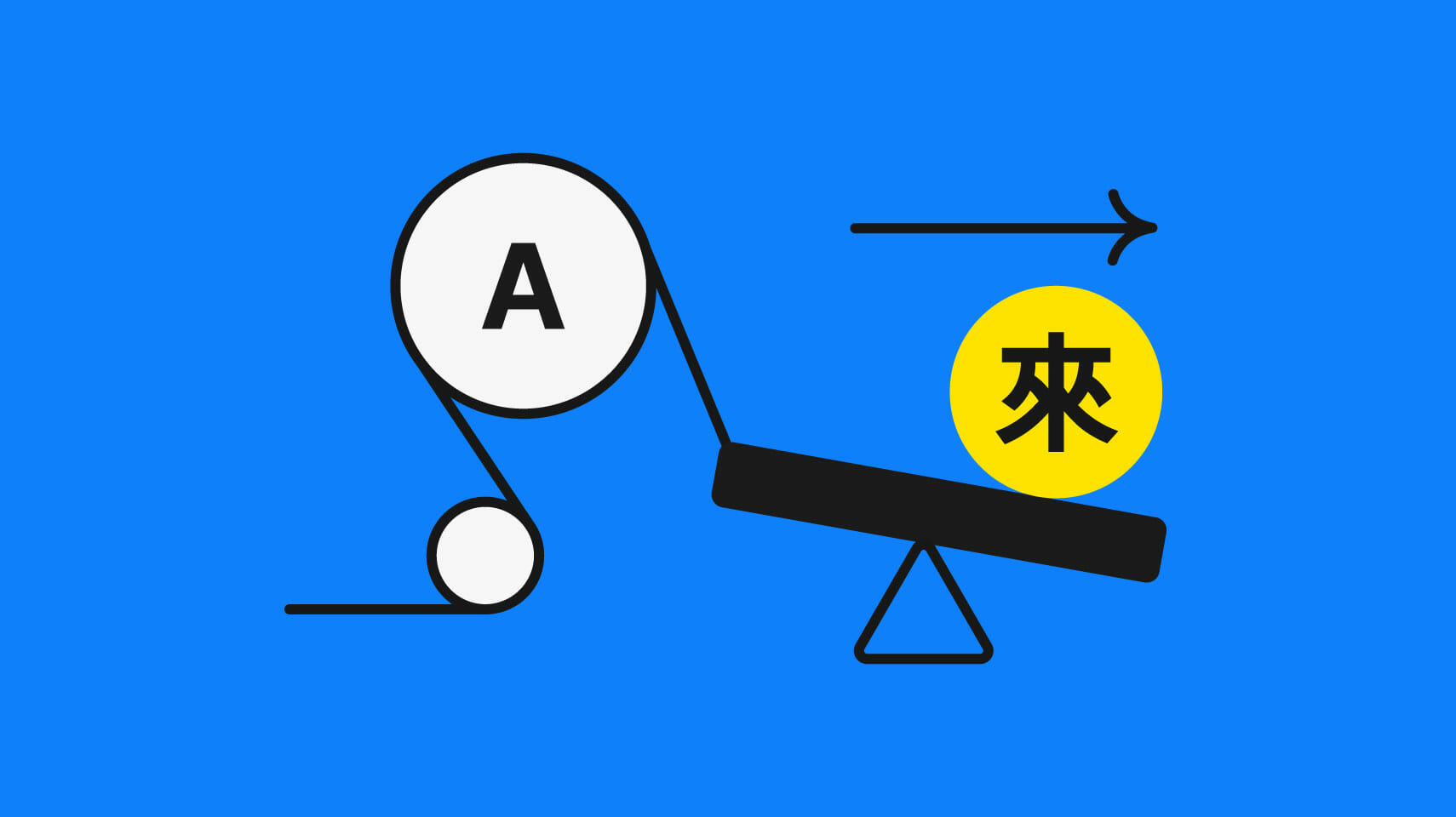
There’s been quite a bit of talk about the effectiveness of machine translation (MT), especially when translating complex or nuanced language expressions. However, thanks to technological advancements, MT tools have become more accurate and, in some cases, even match human translation.
Google Translate has been a pioneer in machine translation technology, but it’s facing more and more competition from rivals like DeepL and Amazon Translate. Let’s explore Google Translate’s accuracy, its performance against competitors, and some best practices for using it effectively.
The evolution of machine translation technology
Since the dawn of ages—the fall of the Babel Tower, for those who believe in it—people have needed to convey ideas in languages they haven’t mastered. This is known as “translation” and stands for the act of rendering text from one language to another.
For that, one would usually rely on someone else, who not only mastered both the “source” (original) language and “target” (translated) language but could also be trusted to translate the meaning of a message as it was originally intended.
This process can be costly and often impractical. Finding reliable translators can be challenging, costs soar, and above all, productivity may be low—but it all used to work for centuries when no alternative solutions were available. The advent of computing tools in the 20th century changed everything.
From statistical models to deep learning in translation technology
Computers brought enormous improvements in translation with their ability to store already translated sentences and match them with new texts to translate. Different computer-assisted translation (CAT) tools were then developed, which significantly improved productivity. Nonetheless, even with heavily involving a CAT tool, matching pairs had to be reviewed in context, and non-matching pairs still needed translation.
Machine translation (MT), which automatically translates non-matching pairs with the help of artificial intelligence, has taken translation capabilities even further.
Also referred to as automatic translation, MT quickly became available to anyone thanks to the internet, and many technology vendors began to offer machine translation services for free. It seemed to solve all the problems posed previously—instant translation done for free.
However, there are still some questions swirling around the use of machine translation software. How best to implement machine translation into translation workflows? How much can you trust the translation produced by a machine? To what extent does MT properly convey the original meaning of the content? In other words, is it accurate?
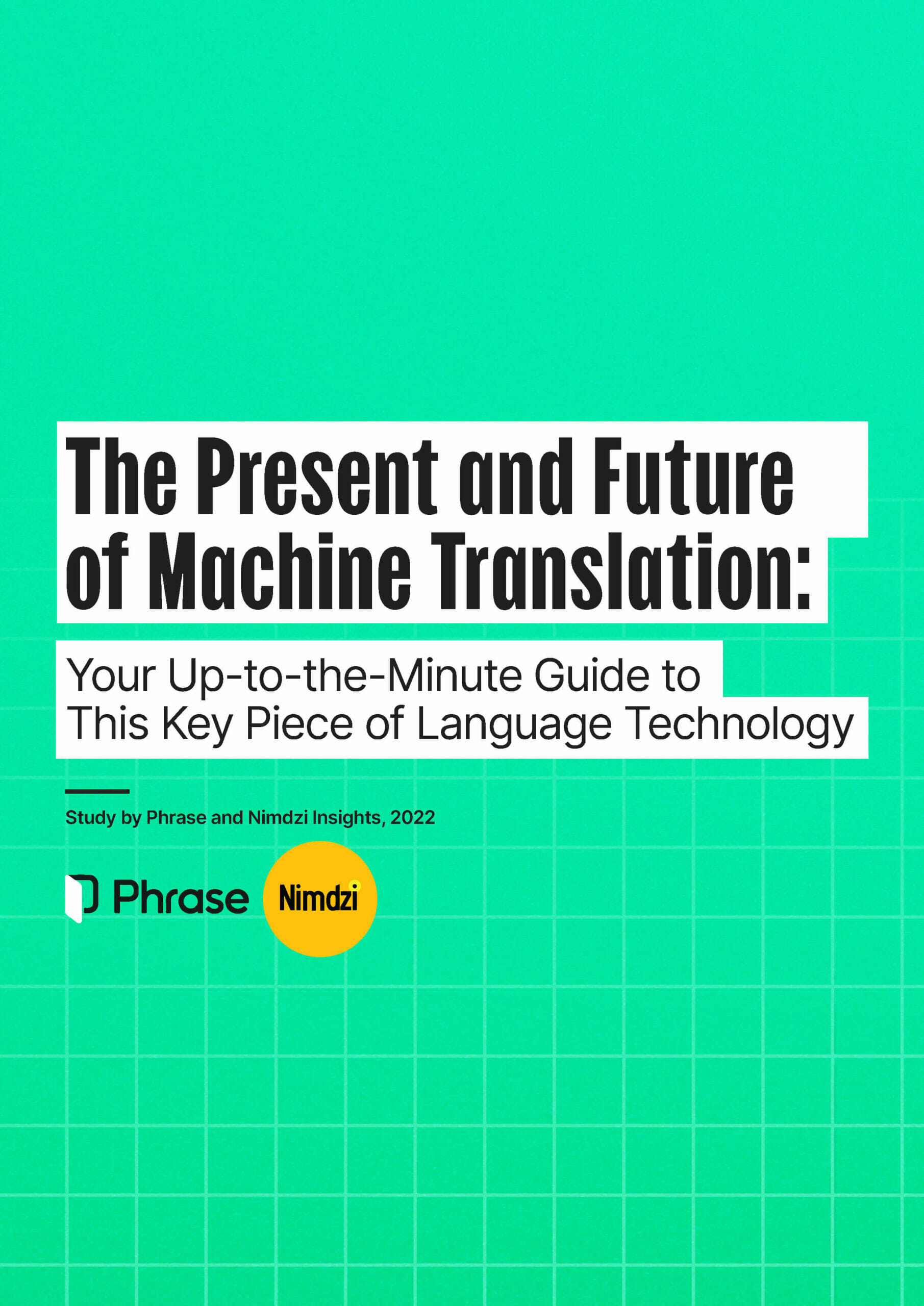
Download for free
Your up-to-the-minute guide to machine translation
Learn about new technologies to improve machine translation output quality, the latest on MT post-editing pricing models, and how to best shop for machine translation.
How Google Translate’s accuracy improved over time
Google Translate has been a key player in MT technology development, steadily improving in accuracy since its launch in 2006. In its early days, it followed rule-based statistical MT methodology, using a set of existing translations (corpora) to translate specific words within sentences.
The quality of statistical machine translation was fairly basic, and developers had to manually define and program what effectively constituted a large set of rules. Due to the low translation quality and high development cost, Google came up with a new MT model in 2016: neural machine translation (NMT).
The rise of neural machine translation
Instead of running a set of predefined rules from the start and inspired by the way human brains work, Google Neural Machine Translation (GNMT) is using neural networks to handle complete sentences as examples of inputs (source text) and outputs (translated text) to predict the translation result.
NMT understands context, can be customized quickly, and detects long-distance dependencies to improve the interpretation of meaning. Its capability to generate translations that resemble human output has made NMT today’s industry standard—with companies like German-based DeepL also competing in the market.
Google Translate’s Transformer model
In 2018, Google Translate released its Transformer model, which introduced attention mechanisms that revolutionized the accuracy of machine translation. By using self-attention layers to better capture context, the model was able to understand and generate translations more accurately than ever before.
Apart from computational performance improvements, the Transformer model has enabled Google Translate to better contextualize words and phrases in a sentence by providing insights into how the neural network “sees” them. It works with a variety of language pairs, and its accuracy varies accordingly.
Google Translate accuracy overview
A 2011 research study found that Google Translate worked well for many European languages but not as accurate for some languages spoken in Asia. The top 10 languages for translation accuracy with English in Google Translate were (from best to worst):
- German
- Afrikaans
- Portuguese
- Spanish
- Danish
- Greek
- Polish
- Hungarian
- Finnish
- Chinese
In 2019, a reevaluation using the same input as the original study showed a 34% improvement in Google Translate’s accuracy.
A more recent study, carried out in 2021 by the UCLA Medical Center, revealed that Google Translate maintained the general meaning in 82.5% of the translations. However, the accuracy levels ranged from 55% to 94%. Here’s a brief overview of the results:
Accuracy levels of Google Translate for different target languages from English source content | |
Spanish | 94% accurate |
Korean | 82.5% accurate |
Mandarin Chinese | 81.7% accurate |
Farsi | 67.5% accurate |
Armenian | 55% accurate |
In general, Google Translate—and most machine translation (MT) systems—excel in languages with abundant training data and high market demand. This typically prioritizes language pairs involving English either as the source or target language, often favoring European languages.
Asian languages, on the other hand, tend to pose challenges because of limited available training data and unique linguistic complexities less typical for European languages. That being said, the performance of MT engines continually undergoes retraining and improvement—leading to changing levels of accuracy and fluency.
How do you assess Google Translate’s performance?
There’s a variety of evaluation metrics for assessing the quality of machine translation engines like Google Translate, with BLEU (BiLingual Evaluation Understudy) and TER (Translation Error Rate) being most widely used.
However, those aren’t the only metrics one can use to evaluate Google Translate’s performance. At Phrase, for example, we have our own proprietary evaluations of how Google Translate and other MT systems perform in terms of accuracy and quality—and we carry them out once a quarter:
In our quarterly Machine Translation Report, we bring together the latest performance data for all of the major MT engines used in real workflows in Phrase TMS, the enterprise-ready translation management system within our Phrase Localization Platform.
We consider 2 key criteria from the start: language pair (source vs target language) and content type (domain).
According to the latest MT Report, the top 3 language pairs used in machine translation projects in Phrase TMS are:
- English-Spanish
- English-French
- English-German
When it comes to content type per language pair, Google Translate has achieved the highest performance scores in the following domains:
- Medical for English-German (91.62)
- Industrial for English-Spanish (86.01)
- Entertainment for English-German (84.34)
The result is based on anonymized machine translation post-editing data collected in a period of 6 months. To gather precise MT quality results, we’ve filtered translation segments to reflect the required post-editing effort as closely as possible: Either MT was used and post-edited, or the linguist translated from scratch despite the availability of MT—suggesting that the MT quality was too low for post-editing.
The result is based on anonymized machine translation post-editing data collected in a period of 6 months. To gather precise MT quality results, we’ve filtered translation segments to reflect the required post-editing effort as closely as possible: Either MT was used and post-edited, or the linguist translated from scratch despite the availability of MT—suggesting that the MT quality was too low for post-editing.
Can you trust Google Translate?
As language and translation are both dynamic categories that intrinsically reflect processes—not static phenomena—accuracy should also be seen as a relative concept. Accuracy in translation will depend on the original intention of the author and the destination of the message. For example, expectations of accuracy in grammar, style, and register for an email will greatly vary from the expectations of accuracy for a novel.
A frequently heard opinion is that Google Translate’s free MT service is accurate enough for most users because they need to translate simple messages—and what matters most is that the audience is able to grasp the sense of it rather than the complete “native” message. It can then be considered accurate enough because expectations are low.
As a rule of thumb, the accuracy of Google Translate’s free MT tool is less consistent when:
- Used as a dictionary to translate single words: Google Translate struggles to produce an accurate result, i.e., as intended by the author, because of the many meanings a single word can have; this is true for English as well as for other widely spoken languages
- Translating familiar expressions that don’t have a direct equivalent in the target language
- Non-verbal expressions are an important part of the message, e.g., when being ironic
- Grammatical rules aren’t properly used in the source language or used differently in the target language, such as the subjunctive mood in English
For business purposes, when a large amount of content needs translation across domains, Google offers its Cloud Translation connected system. Companies can either set it up themselves or rely on a translation management system (TMS) to fully manage it from day one. Cloud Translation offers customization features for domain and context-specific terms as well as the possibility to train custom translation models.
Google’s Cloud Translation offering makes an official statement that it doesn’t use any content submitted for translation for any purpose other than providing the translation service. Nevertheless, it’s unclear how the company uses the information submitted to the free version of Google Translate—or if the data influences business decisions in any way.
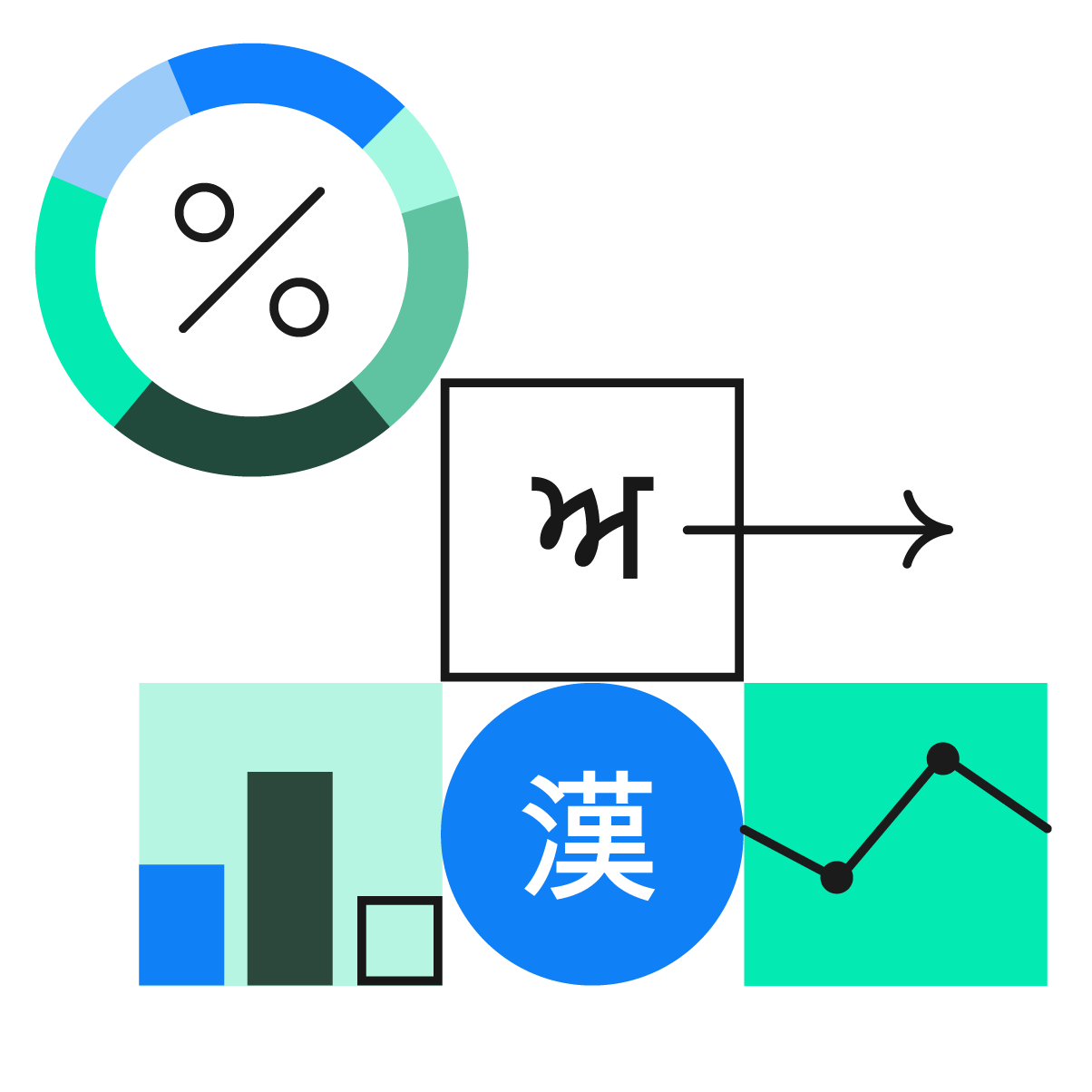
Interactive MT report: Uncover top performers
Find out how leading machine translation engines perform for different content types using the latest data in our quarterly machine translation report.
Does Google Translate have any major competitors?
While Google Translate may be the first name that will pop up when discussing machine translation, there are several competing machine translation software vendors on the market—each of them offering a specific approach to MT.
Here are some of Google Translate’s major competitors to consider when looking for the best machine translation engine:
Amazon Translate
Amazon Translate is part of Amazon Web Service, a subsidiary of Amazon, providing on-demand cloud computing platforms and APIs for both individuals and businesses. It’s based on NMT technology as well.
Amazon Translate supports translation between 75 languages.
DeepL
DeepL is a German-based online MT service that was launched in 2017. It uses a proprietary algorithm with NMT technology and can process DOCX, PPTX, and PDF files while retaining footnotes, formatting, and embedded images.
DeepL supports 26 languages, forming 650 target-to-source combinations.
Systran Translate
Systran is a translation technology company founded in 1968 by a researcher at the California Institute of Technology. It’s one of the first companies to start developing MT software. Its original objective was to improve the translation of Russian into English.
Starting with rule-based MT technology, it developed hybrid RbMT/SMT technology and has since then switched to NMT.
With Systran Translate, you can translate into 50 languages.
Microsoft Translator
Microsoft Translator is a multilingual MT cloud service provided by Microsoft. As part of Microsoft Cognitive Services, it’s integrated with multiple consumer, developer, and enterprise products.
Microsoft Translator supports over 100 languages.
Tencent
Tencent Machine Translation is the main MT offering by Chinese technology giant Tencent. The solution combines both NMT and SMT models.
Tencent Machine Translation supports over 160 different language pairs.
Will Google Translate ever be perfect?
Translation isn’t just about converting words from one language into another. If it were so, a dictionary would be the only necessary tool of the trade, and we all have seen the very poor (and sometimes very funny) results of working that way. This is because a message isn’t only made of words—it also contains context, intention, non-verbal aspects, etc.
That said, Google Translate has been rapidly advancing over the years, but it still can’t do much that human translators can:
- Ask questions
- Understand context
- Catch irony
- Translate creatively
- Make considered choices
- Do research
- Observe consistency
- Guarantee completeness
- Deliberately leave out or include information
- Add glosses/notes
No one knows if and when technology can reach the human level of semantic acuteness, but that’s exactly the goal for many. Quantum computing, for example, aims to increase the number of operations and data that can be processed, so one day it may be able to learn without human interaction and get a better understanding of the creation of language.
How to use Google Translate: Best practices
Google Translate has grown into a strong productivity tool that can save you time and spare you the hassle of looking for a good translator. Generally speaking, you can use Google Translate for texts that don’t need to be perfect in terms of style and consistency, i.e. for anything that won’t make or break your brand:
- Low-visibility or low-traffic content, such as internal documentation, website footers, social media posts for sentiment analysis, etc.
- Repetitive technical content that only needs to be actionable, like instruction manuals, for end-users to access key information to solve a problem
- User-generated content like product reviews, for which consumers generally don’t expect high quality
- Quickly perishable content, like chat or email support messages, customer enquiries, etc.
- Large bulks of content with a short turn-around, such as hundreds of product descriptions that need to go live quickly
- Frequently amended content like feature and information updates
Nevertheless, if you decide to rely exclusively on Google Translate, you may run a considerable risk of your translation lacking important information, meaning, or grammar. To avoid those pitfalls, it’s key to review and adjust your MT output. This process is known as machine translation post-editing (MTPE).
Depending on the level of accuracy you want to achieve, you can apply light or full post-editing. Both approaches will give you the benefits of using MT output while ensuring that your message reaches the intended goal from the start.
As a general guideline, the below cases require machine translation post-editing:
- Product titles: They are highly informative and concise, they tend to contain proper names and polysemous words, and their word order is usually relatively free, which can cause ambiguity.
- Translations between language pairs of dissimilar syntax, like Japanese and Spanish, because the reordering of words and phrases to well-formed sentences becomes more challenging for machine translation engines.
- Product descriptions: They need to be well-crafted and clearly state the product’s features or benefits without room for ambiguity.
- Content of medium visibility that needs to be as accurate as possible: knowledge base, FAQs, alerts, etc.
- Back-end SEO meta information such as image alt texts and captions: While their visibility is low, a human needs to ensure that the target-language keywords are present.
All in all, like all other free MT services, Google Translate’s free MT tool is quite handy when you want to translate relatively simple pieces of text quickly. However, for an accurate translation that properly conveys the original meaning, you’ll want to consider post-editing as the most effective way to use machine translation in the long run.
Unlock the power of machine translation
Discover advanced machine translation management features within our enterprise-ready TMS and create new business opportunities worldwide more quickly and efficiently.