Global business Localization strategy
Ethical AI: The Evolving Role of Localization Managers
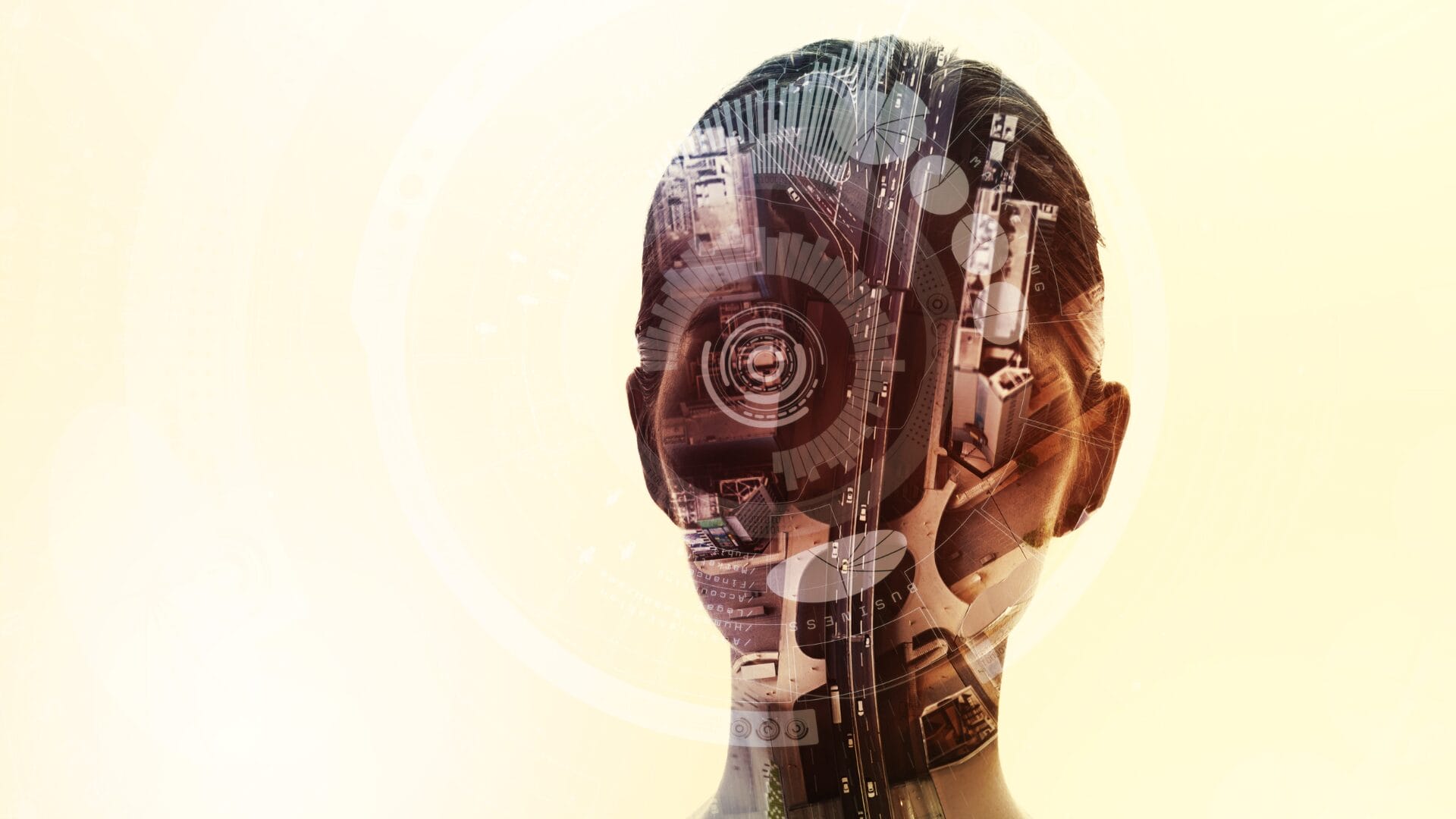
When we talk about artificial intelligence (AI) in localization, there’s a natural tendency to speak about it from an operational angle.
After all, the right use of AI and Large Language Models (LLMs) has been busily revolutionizing high-volume machine translation (MT) for some time. Companies can now handle and translate vast amounts of content quickly and efficiently.
However, there is another major impact that we’re perhaps guilty of dancing around – how exactly will AI impact jobs in our industry.
As more automation and machine-assisted processes become the norm, there’s a natural tendency to worry about people being replaced.
This then leads to other questions: If people are removed from the loop, can we trust AI to handle things? Will the translation quality meet our standards and those of our customers? Should we be worried about AI ‘hallucinations’ in our content?
And the questions don’t stop there.
As AI becomes more prominent, the roles of localization professionals will inevitably shift, but in the majority of cases, this might be the best thing that could happen.
In this article, I’d like to look at how those roles are adapting. More importantly, why the role of humans is so crucial if we’re going to ensure that these technologies are applied ethically, safeguarding both the integrity of translations, and maintaining cultural sensitivity across incredibly diverse and complex markets. Let’s dive in…
The power (and pitfalls) of AI in localization
Efficiency and volume – how AI and LLMs are revolutionizing high-volume translation tasks
Let’s start by thinking about how and why AI and LLMs are being applied currently. One of their main advantages over human translation is the ability to translate very large volumes of content efficiently.
Coupling speed with cost-effectiveness means companies can scale their translation efforts and stick within their budgets. AI also provides a fairly straightforward path to new, often less widely spoken, language pairs, meaning that internationalization is quicker and easier.
Despite these advantages, AI comes with several ethical challenges that need to be addressed so that the systems can be used responsibly:
- Bias in Training Data: Because LLMs utilize such large datasets, any biases in those sets can easily be amplified. In many cases, AI is looking for the most common, repeatable uses of certain terms, so it’s easy to perpetuate bias. This can skew translations, and provide outputs that marginalize or exclude certain groups. Considering the lengths businesses go to ensure cultural sensitivity and relevance, it’s easy to see how this could have a significant impact on brand reputation, and the bottom line.
- Human Bias in AI Use: With the best will in the world, everyone carries conscious and unconscious biases, and it’s all too easy to introduce these into our AI processes. From inadvertently favoring a particular dataset to interpreting outputs based on our own beliefs or cultural background, none of us are immune. If outputs aren’t scrutinized properly, or are applied inappropriately, AI can easily be misapplied.
- Bias For and Against AI: Perhaps most importantly here, there is also a notable bias for or against the use of AI in translation. At one end, company leadership may be clamoring for cost reduction, efficiency and scalability. At the other, localization managers might be concerned about their job and their team being sidelined by new technology, leading to slow adoption and a reluctance to apply AI capabilities in certain areas.
As with most business decisions, it’s all about balance. AI is not, and never has been, designed to fully replace human expertise; rather to augment it and allow localization professionals to focus on more strategic, high-impact tasks.
By addressing these biases and ensuring that AI is used ethically, localization managers can play a vital role in guiding the responsible integration of AI into the industry.
Of course, this is easier said than done. Localization professionals will need to consider which of their existing skills can most readily be employed in AI-led translation processes.
The ethical imperatives in AI-driven localization
Data quality and bias mitigation
As I mentioned earlier, the quality of training data for any LLM is paramount. High quality, and crucially, highly representative datasets are essential to combat bias.
For instance, neural machine translation (NMT) systems often struggle with less widely spoken languages due to the limited availability of high-quality data. This can result in errors and mistranslations that misrepresent these languages and their cultural nuances. This has traditionally been particularly prevalent with many African languages.
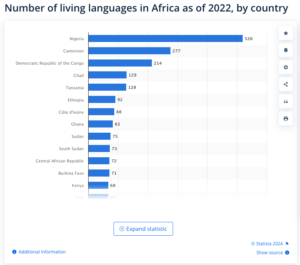
Consider for example, that Nigeria alone has more than 500 languages and dialects, each with a high level of cultural and geographical specificity (and if you want to dive deeper, you might also consider the bias I’m displaying by choosing Africa as a hub of diverse languages).
Culturally sensitive translation is something that is particularly challenging for AI. Context or cultural applications of certain terms change much more frequently than most datasets, leading to translations and applications that might be technically perfect, but could also be inappropriate or even offensive.
As an example, consider a hypothetical situation where AI is being used as part of the content moderation process on a gaming platform.
In the UK, the word ‘Bloody” can be used as a mild curse word for emphasis, but if our user is playing a first-person shooter game, are they actually cursing, or commenting on the amount of pixelated blood that’s spraying around on-screen?
It’s a very minor, and very specific usage, but could well have a compound effect on auto-moderated in-game conversation, blocking content or players for perfectly innocent interactions.
The human element is crucial here, and equipping decision-makers with more culturally aware training processes can make a huge difference.
Culturally diverse businesses often outperform their contemporaries, so it makes sense to build this approach into our AI data as well.
Because we’re talking about a technological problem, we might want to apply a technological solution. In the past, this would have meant “better datasets”, But there is a fundamental difference between earlier neural models and the emerging way in which LLMs are being used.
Neural models were trained from scratch on large data sets. So controlling and manipulating these data sets was very important and consequential. LLMs however, are “pretrained” by the large organizations that create them, so the end user often has little or no control over what information was used for initial training.
However, users do now have much richer methods of control over refining outputs, by interacting with them and adding nuanced instructions and prompts. This instruction could include formal or informal language, changing the tone of voice, avoiding certain words, and more.
This level of control and interactivity is new, and wasn’t possible until quite recently, but again, it very much requires human experts to drive the changes and to evaluate and ensure the results are satisfactory.
Transparency and accountability
The types of AI systems at work in high-volume machine translation can often seem a mystery if you aren’t an expert. If managers and users can’t understand why their AI system is making the choices it makes, they aren’t going to feel comfortable using it – or passing those translations onto users.
Localization professionals are quite right to demand clear, exact explanations from LLM engines and vendors around which datasets and algorithms are being used, and why decisions are being made by these systems.
While training to help understand what is happening ‘under the hood’ can help here, there also needs to be a focus on who trains AI systems, and in particular, how we train them so that they can check and evaluate the output of other AI systems, as well as how users can regularly audit for changes in the levels of bias, and quality rate measurement to spot dips or peaks in accuracy.
If you’re dealing with legal, medical, or indeed any type of personal data, the risks are obviously much higher, and while this is chiefly seen as an engineering issue, any vendors should have clear guidelines around how personal data is anonymized and removed from AI memory. The legal costs of misuse or misapplication can be enormous.
Cultural and contextual sensitivity
It’s hard to underline this enough. AI translation is efficient, but without very clear guidelines and refinements, it is not subtle. Without careful consideration of context, your translations are going to miss the mark in many ways – and not all of them will be expected.
The most obvious example of this might be the literal translation of an idiomatic expression:
“It’s still up in the air”.
Is your project literally on board an airliner right now, or do you simply mean you’re waiting for something to be resolved?
It’s easy to become overly concerned about these things, but on the flipside, getting it right offers huge opportunities to raise engagement and foster loyalty, and this means combining human oversight with large-scale AI powered translation.
(As a delightfully silly example, the French word for “choice” is “Choix”. In the French translation of the Harry Potter books, the famous Sorting Hat is referred to as a “Choixpeau” – a take on “Chapeau”, the French word for hat. It’s often the smallest examples that showcase why and how a human’s insight and ability to interpret can make all the difference.)
The evolving role of localization managers
From tactical to strategic
Localization managers are now strategic business assets. Certainly, there’s still the need to coordinate assets and make sure projects are delivered on time, but the bigger picture is all about balancing the gains offered by AI with the need for high quality, consistent and sensitive translations and transcreation.
Localization managers should ideally be looking at ways to use AI to create more efficient workflows and drive scale, but will also need to be sharing that knowledge by setting operational guidelines for its use.
In practice, this means that a specialist localization professional is in charge of keeping humans in the loop.
In many cases, this means reconfiguring processes and ensuring that translation teams are able to review and optimize AI-generated content regularly.
It also means developing new methods to assess how effectively teams improve or correct flagged content. In this new era, monitoring dashboards and analysis tools become crucial.
Similar to the ways Engineering and Marketing teams work, the modern localization team needs a set of tools and processes that adopt the ‘kaizen’ way of working – a method that consistently looks for ways to optimize both processes and outputs.
Localization managers as educators
The localization team is now a hub of knowledge, and education is fast becoming one of their primary responsibilities.
While it may seem we’re reaching a saturation point with AI, in practice we are far from it, and knowledge is often very unevenly distributed across the business. Localization managers are perfectly positioned to inform and educate decision-makers on the capabilities and limitations of AI.
This may seem like yet more work being heaped on to localization managers’ plates, but luckily, there are standard approaches that can help here.
Organizing training programs and ‘lunch and learn’ sessions for internal teams, working with marketing to develop more informative assets for clients, and sharing reporting to help set reasonable, contextual expectations for AI performance, backed up by solid numbers.
This increased engagement with the broader business can also help localization teams emphasize just how important it is to keep human oversight and use it in conjunction with AI to get better results. After all, if you are the ones sharing all the knowledge, how can you be replaced?
Beyond being the go-to source of knowledge, there are also ways localization teams can showcase their value during the initial set-up and ongoing running of AI technologies.
Three ways localization teams can make sure AI is used ethically
Build ethical guidelines
Every new technology needs a set of robust guidelines before rollout, and while a good vendor will have these in place, it pays to pay close attention and plot out clear guidance for areas like data quality, bias reduction, and translation training to accommodate cultural nuance.
Strong frameworks should always include the need for human oversight. While this oversight will need to be more robust for materials and translations where more nuance is required, it’s important to have regular checks and balances in place wherever you employ AI to scale translations. In the past, there have been several high-profile examples of seemingly simple applications going rogue, or being open to interference from outside actors. Always remember that AI is never a ‘set and forget’ solution, but an active and evolving system.
By setting clear rules, localization managers can ensure that AI supports, rather than undermines, industry ethics.
Ongoing monitoring and refinement
Speaking of continuous involvement, remember to plan in regular audits of your AI input and outputs. These are absolutely key to spotting and addressing biases and errors that can creep in. As more information is provided to an LLM, so the risk of bias increases, so make sure you are performing regular checks.
Localization teams can use both AI and human experts to review translation processes regularly. Automated tools can flag issues in AI-generated translations, while human experts fine-tune for accuracy and cultural fit. This approach will also keep improving AI, making it more reliable over time.
Transparency in AI
Finally, let’s think about transparency in more detail.
From a customer-facing perspective, companies and organizations need to be clear about how and where they use AI, and to be honest about the potential for bias and errors in results. As in other areas of the business, being open about risks and the steps being taken to limit them is a key part of building trust with stakeholders and customers. This also provides an opportunity where the localization team can showcase their expertise in both the benefits and risks of AI—key strategic areas where the team should have a voice.
As an example, including being transparent about where AI is used can help set clear expectations for users. It’s equally important to communicate internally about which data is used and how it has been prepared before it is used to train AI systems.
Clean data is an imperative, and while it means more work up front, it will always lead to better results. By focusing on transparency, localization teams can promote a more ethical and responsible use of AI.
Ways Phrase can help address ethical issues
One of the main drivers behind Phrase’s own Quality Performance Scores (QPS) is to make translation quality more transparent. By breaking down how scores are calculated using the MQM 2.0 framework, it gives localization managers greater insight into the inner workings of AI translations, and is an opportunity to spot changes in quality, accuracy and bias.
Similarly, Auto LQA acts like automatic pre-translation, handling the initial quality check so that linguists can focus on refining and validating the results—ultimately saving time and effort.
For dispersed localization teams, centralizing translation tasks through a single platform like Phrase can help promote transparency, helping teams across different locations stay aligned and informed. This visibility reduces errors and miscommunication, ensuring projects remain consistent and high-quality.
Conclusion
The integration of AI and large language models (LLMs) into localization has brought both exciting opportunities and important ethical questions. As AI reshapes high-volume translation tasks, it’s crucial to keep standards high in areas like data quality, transparency, and cultural sensitivity. Localization managers now play a bigger role, moving from day-to-day tasks to overseeing how AI is used responsibly and effectively.
Key ethical issues—like bias in AI training data, the need for human oversight, and the importance of being transparent—are critical for the ethical use of AI in localization. Using AI responsibly means recognizing that it should enhance human expertise, not replace it. AI should be a tool that supports localization, without compromising quality or cultural respect.
Localization managers are leading this change. Their active role in shaping AI practices, educating teams, and setting ethical standards will ensure that AI remains a helpful resource in the industry. By taking these steps, they’ll help create a future where AI-powered localization not only meets the needs of global communication but also respects the diverse cultures and languages involved.